AI Breakdown
The podcast where we use AI to breakdown the recent AI papers and provide simplified explanations of intricate AI topics for educational purposes. The content presented here is generated automatically by utilizing LLM and text to speech technologies. While every effort is made to ensure accuracy, any potential misrepresentations or inaccuracies are unintentional due to evolving technology. We value your feedback to enhance our podcast and provide you with the best possible learning experience.
Episodes
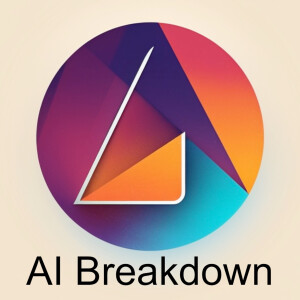
Tuesday Aug 06, 2024
Tuesday Aug 06, 2024
In this episode, we discuss Cycle3D: High-quality and Consistent Image-to-3D Generation via Generation-Reconstruction Cycle by Zhenyu Tang, Junwu Zhang, Xinhua Cheng, Wangbo Yu, Chaoran Feng, Yatian Pang, Bin Lin, Li Yuan. Recent 3D large reconstruction models often generate low-quality and inconsistent multi-view images, which harm the final 3D output. To resolve this, the proposed Cycle3D framework integrates a 2D diffusion-based generation module and a 3D reconstruction module to iteratively enhance texture quality and multi-view consistency. Experiments show that Cycle3D outperforms state-of-the-art methods in creating high-quality and consistent 3D content.
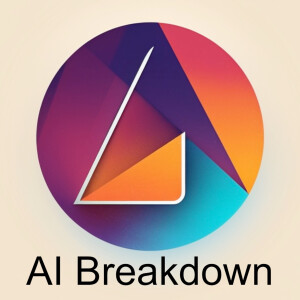
Tuesday Aug 06, 2024
Tuesday Aug 06, 2024
In this episode, we discuss Towards Achieving Human Parity on End-to-end Simultaneous Speech Translation via LLM Agent by Shanbo Cheng, Zhichao Huang, Tom Ko, Hang Li, Ningxin Peng, Lu Xu, Qini Zhang. The paper introduces CLASI, a high-quality and human-like Simultaneous Speech Translation (SiST) system inspired by professional interpreters' strategies to balance translation quality and latency. Utilizing a multi-modal retrieving module and Large Language Models (LLMs), CLASI significantly outperforms other systems, especially in challenging real-world scenarios. Evaluated using the valid information proportion (VIP) metric, CLASI achieves impressive results compared to state-of-the-art systems, with VIP scores of 81.3% for Chinese-to-English and 78.0% for English-to-Chinese translations.
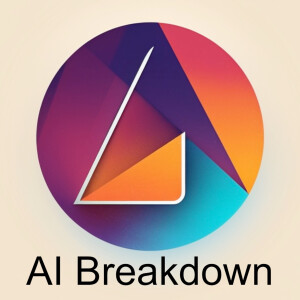
Wednesday Jul 31, 2024
Wednesday Jul 31, 2024
In this episode, we discuss Graph-enhanced Large Language Models in Asynchronous Plan Reasoning by Fangru Lin, Emanuele La Malfa, Valentin Hofmann, Elle Michelle Yang, Anthony Cohn, Janet B. Pierrehumbert. The paper investigates how well large language models (LLMs) like GPT-4 and LLaMA-2 handle reasoning about asynchronous plans and finds that they perform poorly without visual aids. It introduces a new technique, Plan Like a Graph (PLaG), which integrates graphs with language prompts, significantly improving model performance. Despite this improvement, the study highlights the limitations of LLMs when dealing with complex tasks, underscoring the challenges of using them as autonomous agents.
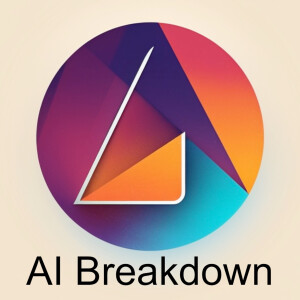
Tuesday Jul 30, 2024
Tuesday Jul 30, 2024
In this episode, we discuss LazyLLM: Dynamic Token Pruning for Efficient Long Context LLM Inference by Qichen Fu, Minsik Cho, Thomas Merth, Sachin Mehta, Mohammad Rastegari, Mahyar Najibi. The paper introduces LazyLLM, a method that selectively computes only the essential token's Key-Value (KV) cache for next token prediction during the prefilling and decoding stages of transformer-based language models to address the bottleneck caused by long prompts. Unlike static pruning approaches, LazyLLM dynamically adapts which tokens to consider at each generation step. This method significantly accelerates the generation process without sacrificing accuracy, as demonstrated in experiments like the multi-document question-answering task with LLama 2 7B model, achieving a 2.34× speedup.
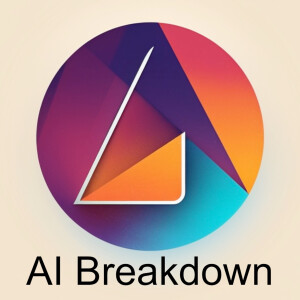
Monday Jul 29, 2024
Monday Jul 29, 2024
In this episode, we discuss OutfitAnyone: Ultra-high Quality Virtual Try-On for Any Clothing and Any Person by Ke Sun, Jian Cao, Qi Wang, Linrui Tian, Xindi Zhang, Lian Zhuo, Bang Zhang, Liefeng Bo, Wenbo Zhou, Weiming Zhang, Daiheng Gao. Virtual Try-On (VTON) technology faces challenges in generating high-fidelity and consistent images. While existing diffusion models struggle with control in VTON scenarios, OutfitAnyone uses a two-stream conditional diffusion model to overcome these issues, achieving lifelike results and scalability across various scenarios. This method effectively handles garment deformation and adapts to different poses, body shapes, and image types, making it suitable for real-world applications.
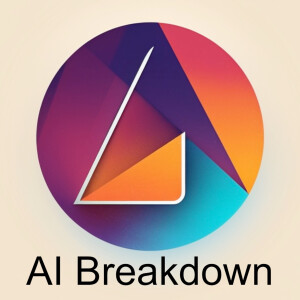
Friday Jul 26, 2024
Friday Jul 26, 2024
In this episode, we discuss DetToolChain: A New Prompting Paradigm to Unleash Detection Ability of MLLM by Yixuan Wu, Yizhou Wang, Shixiang Tang, Wenhao Wu, Tong He, Wanli Ouyang, Philip Torr, Jian Wu. DetToolChain introduces a prompting toolkit and a Chain-of-Thought methodology to enhance zero-shot object detection capabilities in multimodal large language models like GPT-4V and Gemini. The toolkit employs precise detection strategies and tools such as zooming, overlaying rulers, and scene graphs to help the models focus and infer better. Experimental results demonstrate significant performance improvements in various detection tasks, surpassing state-of-the-art methods considerably.
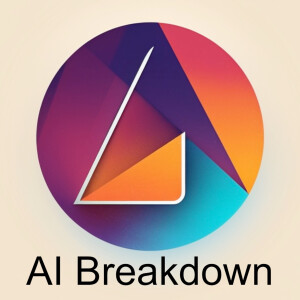
Tuesday Jul 23, 2024
Tuesday Jul 23, 2024
In this episode, we discuss Conditioned Language Policy: A General Framework for Steerable Multi-Objective Finetuning by Kaiwen Wang, Rahul Kidambi, Ryan Sullivan, Alekh Agarwal, Christoph Dann, Andrea Michi, Marco Gelmi, Yunxuan Li, Raghav Gupta, Avinava Dubey, Alexandre Ramé, Johan Ferret, Geoffrey Cideron, Le Hou, Hongkun Yu, Amr Ahmed, Aranyak Mehta, Léonard Hussenot, Olivier Bachem, Edouard Leurent. The paper presents Conditioned Language Policies (CLP), a framework for finetuning language models to balance multiple conflicting objectives. CLP leverages multi-task training and parameter-efficient finetuning to allow a single model to navigate trade-offs between objectives during inference. Experiments show that CLP outperforms existing methods, making it a superior approach for creating steerable and flexible language models.
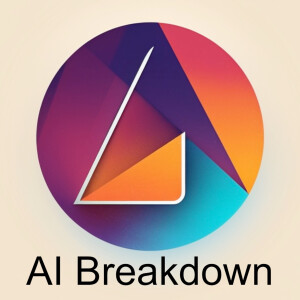
Monday Jul 22, 2024
Monday Jul 22, 2024
In this episode, we discuss Chameleon: Mixed-Modal Early-Fusion Foundation Models by Chameleon Team. The paper introduces Chameleon, a family of models designed for seamless understanding and generating both images and text in any sequence. It achieves state-of-the-art performance in several tasks, including image captioning and text generation, and demonstrates competence in mixed-modal outputs. Notably, Chameleon is competitive with or superior to larger models like Gemini Pro and GPT-4V in various evaluations, highlighting its significance in multimodal document processing.
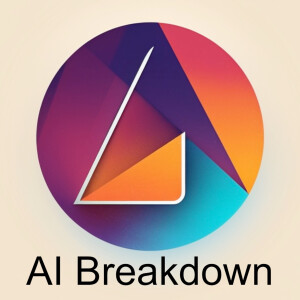
Thursday Jul 18, 2024
Thursday Jul 18, 2024
In this episode, we discuss Goldfish: Vision-Language Understanding of Arbitrarily Long Videos by Kirolos Ataallah, Xiaoqian Shen, Eslam Abdelrahman, Essam Sleiman, Mingchen Zhuge, Jian Ding, Deyao Zhu, Jürgen Schmidhuber, Mohamed Elhoseiny. The paper introduces Goldfish, a methodology designed to efficiently comprehend videos of any length by employing a retrieval mechanism that selects top-k relevant video clips for processing. To evaluate its effectiveness, the authors present the TVQA-long benchmark aimed at long video understanding and demonstrate significant improvements over existing methods, achieving a 41.78% accuracy rate. Additionally, their MiniGPT4-Video model also excels in short video comprehension, outperforming current state-of-the-art methods on multiple benchmarks.
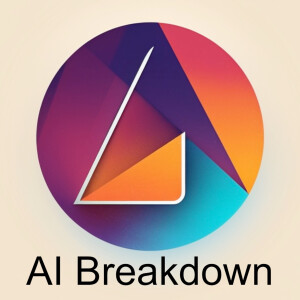
Wednesday Jul 17, 2024
Wednesday Jul 17, 2024
In this episode, we discuss Masked Generative Video-to-Audio Transformers with Enhanced Synchronicity by Santiago Pascual, Chunghsin Yeh, Ioannis Tsiamas, Joan Serrà. The paper introduces MaskVAT, a video-to-audio generative model that utilizes a masked generative model alongside a high-quality general audio codec to achieve superior audio quality, semantic matching, and temporal synchronization. MaskVAT effectively addresses the synchronization issues in previous V2A models without compromising on audio quality. Empirical results demonstrate its capability to generate well-synchronized and high-quality audio that aligns with visual actions, competing with state-of-the-art non-codec generative models.
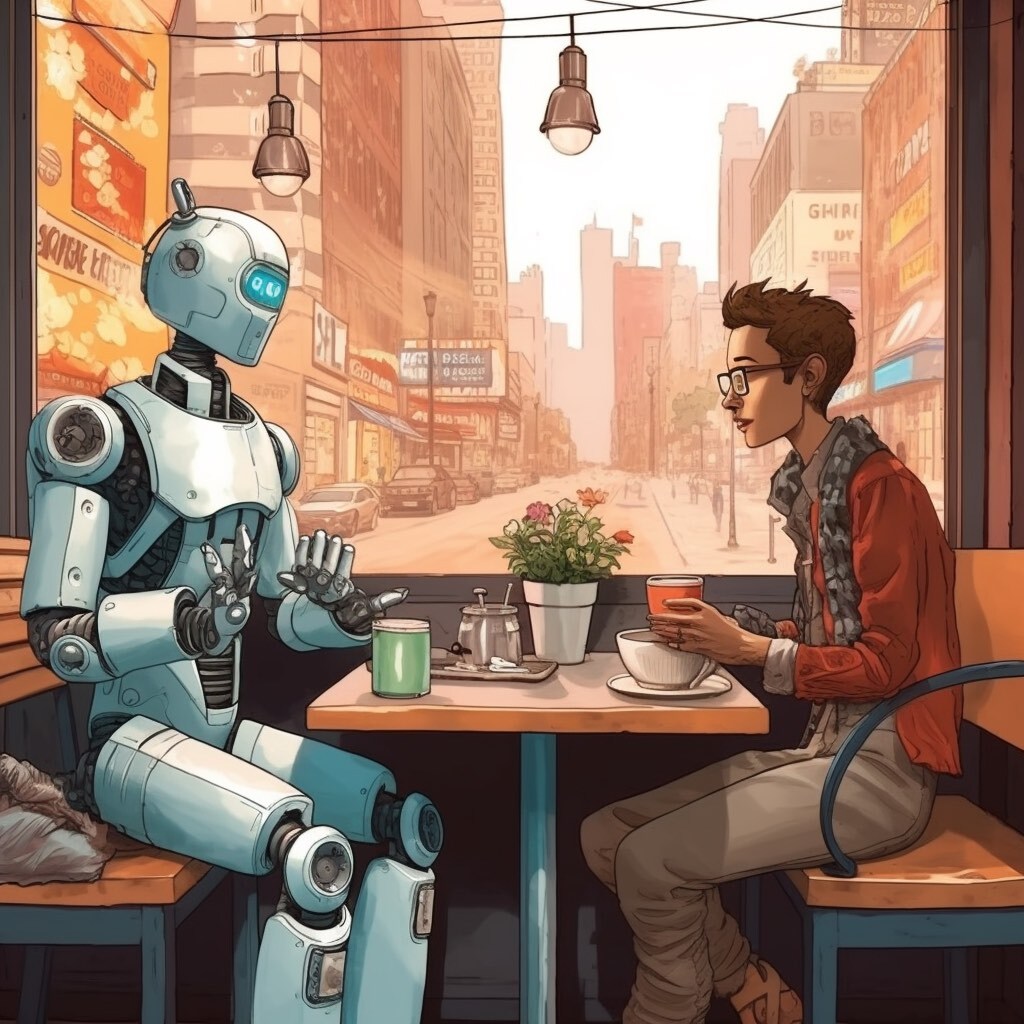
Leverage AI to learn AI
Welcome to the AI Breakdown podcast, where we leverage the power of artificial intelligence to break down recent AI papers and provide simplified explanations of intricate AI topics for educational purposes. We're delighted to have you join us on this exciting journey into the world of artificial intelligence. Our goal is to make complex AI concepts accessible to everyone, and we achieve this by utilizing advanced AI technologies.
Hosts and Ownership: AI Breakdown is under the ownership and management of Megan Maghami and Ramin (Ray) Mehran. Although Megan and Ray lend their voices to the podcast, the content and audio are produced through automated means. Prior to publication, they carefully review the episodes created by AI. They leverage advanced AI technologies, including cutting-edge Large Language Models (LLM) and Text-to-Speech (TTS) systems, to generate captivating episodes. By harnessing these ingenious tools, they deliver enlightening explanations and in-depth analyses on various AI subjects.
Enhancing Your Learning Experience: Your feedback and engagement are crucial to us as we strive to enhance the podcast and provide you with the best possible learning experience. We encourage you to share your thoughts, suggestions, and questions related to our episodes. Together, we can build a vibrant community of AI enthusiasts, learners, and experts, fostering collaboration and knowledge sharing.
Technical Details and Episode Archives: For those interested in the technical aspects behind our AI-generated content, we will provide further insights in upcoming blog posts. Additionally, we will regularly update the blog with published episodes of the AI Breakdown podcast, ensuring convenient access to all our educational resources.