AI Breakdown
The podcast where we use AI to breakdown the recent AI papers and provide simplified explanations of intricate AI topics for educational purposes. The content presented here is generated automatically by utilizing LLM and text to speech technologies. While every effort is made to ensure accuracy, any potential misrepresentations or inaccuracies are unintentional due to evolving technology. We value your feedback to enhance our podcast and provide you with the best possible learning experience.
Episodes
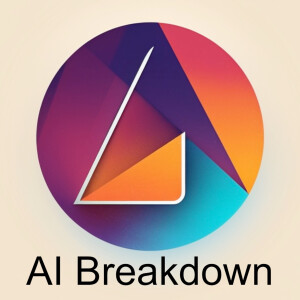
13 hours ago
13 hours ago
In this episode, we discuss DetToolChain: A New Prompting Paradigm to Unleash Detection Ability of MLLM by Yixuan Wu, Yizhou Wang, Shixiang Tang, Wenhao Wu, Tong He, Wanli Ouyang, Philip Torr, Jian Wu. DetToolChain introduces a prompting toolkit and a Chain-of-Thought methodology to enhance zero-shot object detection capabilities in multimodal large language models like GPT-4V and Gemini. The toolkit employs precise detection strategies and tools such as zooming, overlaying rulers, and scene graphs to help the models focus and infer better. Experimental results demonstrate significant performance improvements in various detection tasks, surpassing state-of-the-art methods considerably.
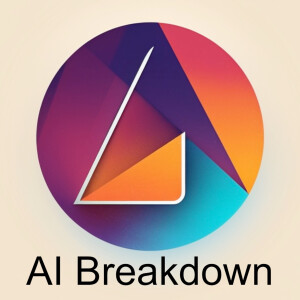
4 days ago
4 days ago
In this episode, we discuss Conditioned Language Policy: A General Framework for Steerable Multi-Objective Finetuning by Kaiwen Wang, Rahul Kidambi, Ryan Sullivan, Alekh Agarwal, Christoph Dann, Andrea Michi, Marco Gelmi, Yunxuan Li, Raghav Gupta, Avinava Dubey, Alexandre Ramé, Johan Ferret, Geoffrey Cideron, Le Hou, Hongkun Yu, Amr Ahmed, Aranyak Mehta, Léonard Hussenot, Olivier Bachem, Edouard Leurent. The paper presents Conditioned Language Policies (CLP), a framework for finetuning language models to balance multiple conflicting objectives. CLP leverages multi-task training and parameter-efficient finetuning to allow a single model to navigate trade-offs between objectives during inference. Experiments show that CLP outperforms existing methods, making it a superior approach for creating steerable and flexible language models.
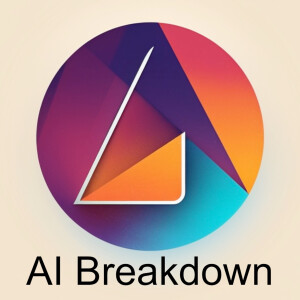
5 days ago
5 days ago
In this episode, we discuss Chameleon: Mixed-Modal Early-Fusion Foundation Models by Chameleon Team. The paper introduces Chameleon, a family of models designed for seamless understanding and generating both images and text in any sequence. It achieves state-of-the-art performance in several tasks, including image captioning and text generation, and demonstrates competence in mixed-modal outputs. Notably, Chameleon is competitive with or superior to larger models like Gemini Pro and GPT-4V in various evaluations, highlighting its significance in multimodal document processing.
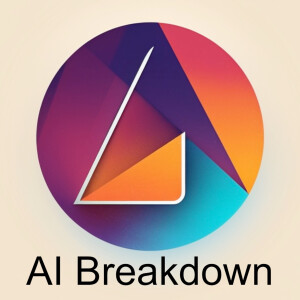
Thursday Jul 18, 2024
Thursday Jul 18, 2024
In this episode, we discuss Goldfish: Vision-Language Understanding of Arbitrarily Long Videos by Kirolos Ataallah, Xiaoqian Shen, Eslam Abdelrahman, Essam Sleiman, Mingchen Zhuge, Jian Ding, Deyao Zhu, Jürgen Schmidhuber, Mohamed Elhoseiny. The paper introduces Goldfish, a methodology designed to efficiently comprehend videos of any length by employing a retrieval mechanism that selects top-k relevant video clips for processing. To evaluate its effectiveness, the authors present the TVQA-long benchmark aimed at long video understanding and demonstrate significant improvements over existing methods, achieving a 41.78% accuracy rate. Additionally, their MiniGPT4-Video model also excels in short video comprehension, outperforming current state-of-the-art methods on multiple benchmarks.
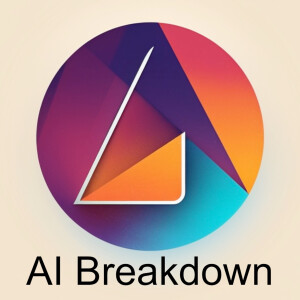
Wednesday Jul 17, 2024
Wednesday Jul 17, 2024
In this episode, we discuss Masked Generative Video-to-Audio Transformers with Enhanced Synchronicity by Santiago Pascual, Chunghsin Yeh, Ioannis Tsiamas, Joan Serrà. The paper introduces MaskVAT, a video-to-audio generative model that utilizes a masked generative model alongside a high-quality general audio codec to achieve superior audio quality, semantic matching, and temporal synchronization. MaskVAT effectively addresses the synchronization issues in previous V2A models without compromising on audio quality. Empirical results demonstrate its capability to generate well-synchronized and high-quality audio that aligns with visual actions, competing with state-of-the-art non-codec generative models.
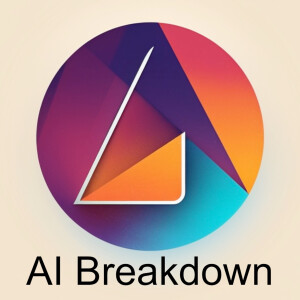
Monday Jul 15, 2024
Monday Jul 15, 2024
In this episode, we discuss Human-like Episodic Memory for Infinite Context LLMs by Zafeirios Fountas, Martin A Benfeghoul, Adnan Oomerjee, Fenia Christopoulou, Gerasimos Lampouras, Haitham Bou-Ammar, Jun Wang. The paper introduces EM-LLM, an approach that enhances large language models (LLMs) by incorporating principles of human episodic memory and event cognition, enabling them to manage extensive contexts efficiently. EM-LLM uses Bayesian surprise and graph-theoretic boundary refinement to organize token sequences into episodic events and employs a two-stage memory process for effective retrieval. Experiments demonstrate that EM-LLM outperforms existing models on various tasks, showing significant improvement, and aligning well with human event perception, suggesting potential for interdisciplinary AI and cognitive science research.
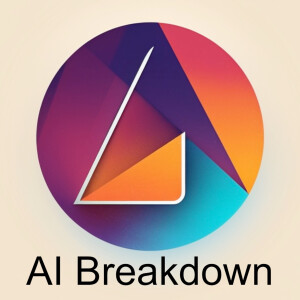
Friday Jul 12, 2024
Friday Jul 12, 2024
In this episode, we discuss Learning to (Learn at Test Time): RNNs with Expressive Hidden States by Yu Sun, Xinhao Li, Karan Dalal, Jiarui Xu, Arjun Vikram, Genghan Zhang, Yann Dubois, Xinlei Chen, Xiaolong Wang, Sanmi Koyejo, Tatsunori Hashimoto, Carlos Guestrin. The paper introduces Test-Time Training (TTT) layers, a new type of sequence modeling layer combining the efficiency of RNNs with the long-context performance of self-attention mechanisms. TTT layers make use of a machine learning model as their hidden state, updated through self-supervised learning iterations even on test sequences. The proposed TTT-Linear and TTT-MLP models demonstrate competitive or superior performance to both advanced Transformers and modern RNNs like Mamba, with TTT-Linear proving more efficient in certain long-context scenarios.
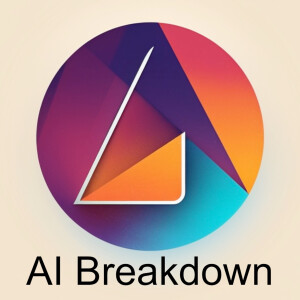
Thursday Jul 11, 2024
Thursday Jul 11, 2024
In this episode, we discuss Graph-Based Captioning: Enhancing Visual Descriptions by Interconnecting Region Captions by Yu-Guan Hsieh, Cheng-Yu Hsieh, Shih-Ying Yeh, Louis Béthune, Hadi Pour Ansari, Pavan Kumar Anasosalu Vasu, Chun-Liang Li, Ranjay Krishna, Oncel Tuzel, Marco Cuturi. The paper introduces a new annotation strategy termed graph-based captioning (GBC) that uses labelled graph structures to describe images more richly than plain text. GBC combines object detection and dense captioning to create a hierarchical graph of nodes and edges detailing entities and their relationships. The authors demonstrate the effectiveness of GBC by creating a large dataset, GBC10M, which significantly improves performance in vision-language models and propose a novel attention mechanism to utilize the graph's structure for further benefits.
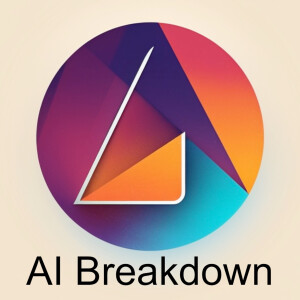
Tuesday Jul 09, 2024
Tuesday Jul 09, 2024
In this episode, we discuss Evaluating Human Alignment and Model Faithfulness of LLM Rationale by Mohsen Fayyaz, Fan Yin, Jiao Sun, Nanyun Peng. The paper investigates how effectively large language models (LLMs) can explain their decisions through rationales extracted from input texts. It compares two types of rationale extraction methods—attribution-based and prompting-based—finding that prompting-based rationales better align with human-annotated rationales. The study also explores the faithfulness limitations of prompting-based methods and shows that fine-tuning models on specific datasets can improve the faithfulness of both rationale extraction approaches.
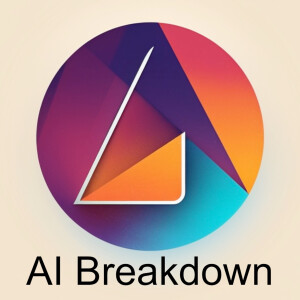
Monday Jul 08, 2024
Monday Jul 08, 2024
In this episode, we discuss Detection and Measurement of Syntactic Templates in Generated Text by Chantal Shaib, Yanai Elazar, Junyi Jessy Li, Byron C. Wallace. The paper investigates syntactic features in text generated by large language models (LLMs), revealing higher rates of templated text in these models compared to human-generated text. It finds that a significant portion of these templates originates from pre-training data and remain unchanged during fine-tuning. The study demonstrates that syntactic templates can distinguish between different models and tasks, and serves as an effective tool for evaluating style memorization in LLMs.
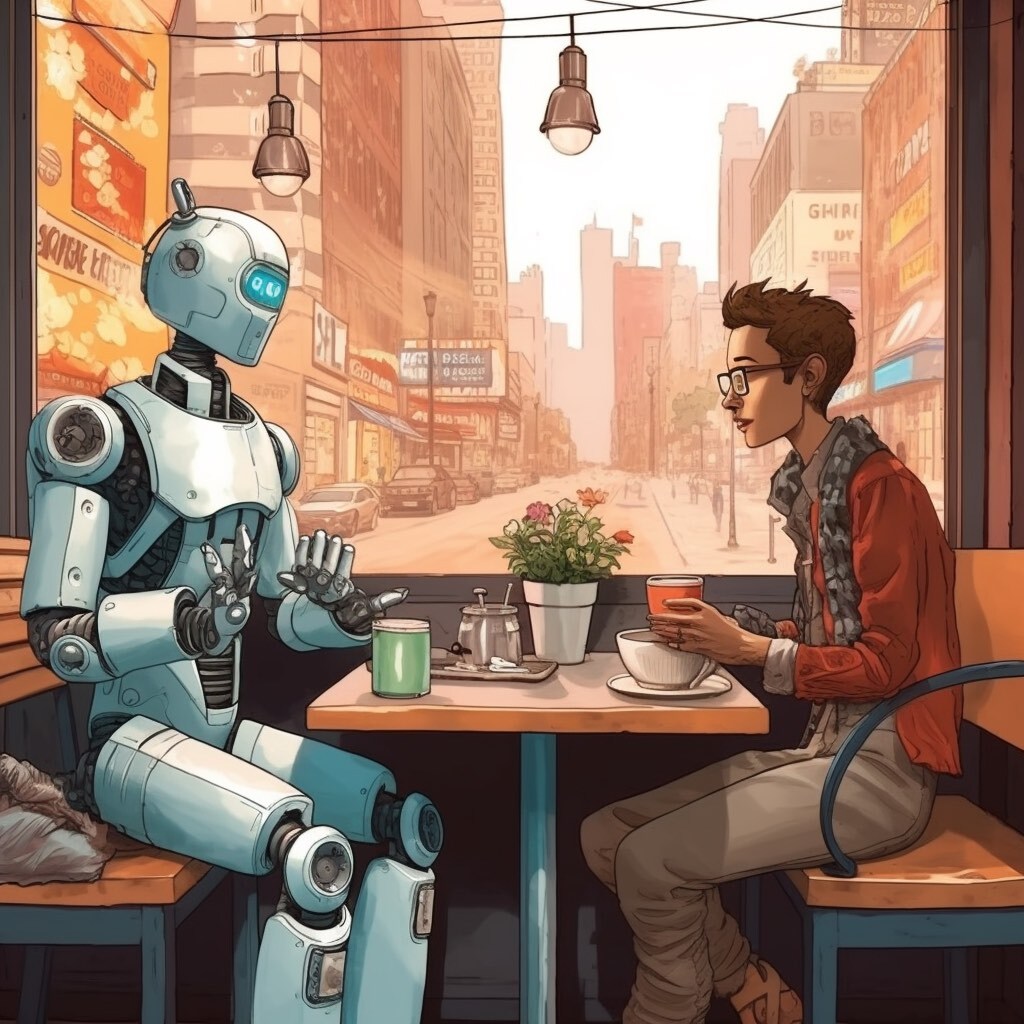
Leverage AI to learn AI
Welcome to the AI Breakdown podcast, where we leverage the power of artificial intelligence to break down recent AI papers and provide simplified explanations of intricate AI topics for educational purposes. We're delighted to have you join us on this exciting journey into the world of artificial intelligence. Our goal is to make complex AI concepts accessible to everyone, and we achieve this by utilizing advanced AI technologies.
Hosts and Ownership: AI Breakdown is under the ownership and management of Megan Maghami and Ramin (Ray) Mehran. Although Megan and Ray lend their voices to the podcast, the content and audio are produced through automated means. Prior to publication, they carefully review the episodes created by AI. They leverage advanced AI technologies, including cutting-edge Large Language Models (LLM) and Text-to-Speech (TTS) systems, to generate captivating episodes. By harnessing these ingenious tools, they deliver enlightening explanations and in-depth analyses on various AI subjects.
Enhancing Your Learning Experience: Your feedback and engagement are crucial to us as we strive to enhance the podcast and provide you with the best possible learning experience. We encourage you to share your thoughts, suggestions, and questions related to our episodes. Together, we can build a vibrant community of AI enthusiasts, learners, and experts, fostering collaboration and knowledge sharing.
Technical Details and Episode Archives: For those interested in the technical aspects behind our AI-generated content, we will provide further insights in upcoming blog posts. Additionally, we will regularly update the blog with published episodes of the AI Breakdown podcast, ensuring convenient access to all our educational resources.