AI Breakdown
The podcast where we use AI to breakdown the recent AI papers and provide simplified explanations of intricate AI topics for educational purposes. The content presented here is generated automatically by utilizing LLM and text to speech technologies. While every effort is made to ensure accuracy, any potential misrepresentations or inaccuracies are unintentional due to evolving technology. We value your feedback to enhance our podcast and provide you with the best possible learning experience.
Episodes
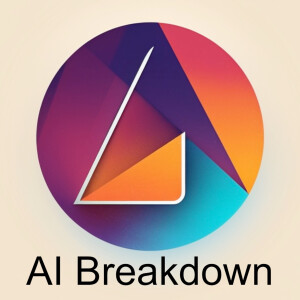
Thursday Feb 27, 2025
Thursday Feb 27, 2025
In this episode, we discuss PANDAS: Improving Many-shot Jailbreaking via Positive Affirmation, Negative Demonstration, and Adaptive Sampling by Avery Ma, Yangchen Pan, Amir-massoud Farahmand. The paper introduces PANDAS, a hybrid technique that enhances many-shot jailbreaking by altering fabricated dialogues with positive affirmations, negative demonstrations, and optimized adaptive sampling tailored to specific prompts. Experimental results on AdvBench and HarmBench using advanced large language models show that PANDAS significantly outperforms existing baseline methods in scenarios involving long input contexts. Additionally, an attention analysis highlights how PANDAS exploits long-context vulnerabilities, providing deeper insights into the mechanics of many-shot jailbreaking.
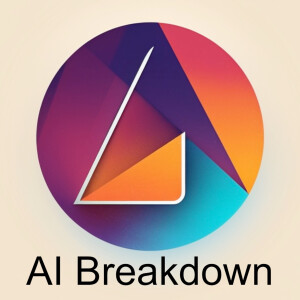
Monday Feb 24, 2025
Monday Feb 24, 2025
In this episode, we discuss VidCRAFT3: Camera, Object, and Lighting Control for Image-to-Video Generation by Sixiao Zheng, Zimian Peng, Yanpeng Zhou, Yi Zhu, Hang Xu, Xiangru Huang, Yanwei Fu. The paper presents VidCRAFT3, a new framework for image-to-video generation that allows simultaneous control over camera motion, object movement, and lighting direction. It addresses previous limitations by introducing the Spatial Triple-Attention Transformer, which effectively decouples and integrates lighting, text, and image inputs. This innovative approach enhances the precision and versatility of controlling multiple visual elements in generated videos.
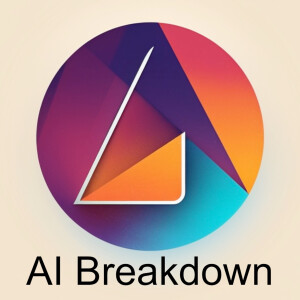
Friday Feb 21, 2025
Friday Feb 21, 2025
In this episode, we discuss Heuristically Adaptive Diffusion-Model Evolutionary Strategy by Benedikt Hartl, Yanbo Zhang, Hananel Hazan, Michael Levin. The paper explores the connection between diffusion models and evolutionary algorithms, highlighting that both generate high-quality samples through iterative refinement of random initial states. By integrating deep learning-based diffusion models into evolutionary processes, the authors enhance convergence efficiency and maintain diversity by leveraging improved memory and refined sample generation. This framework advances evolutionary optimization by providing greater flexibility, precision, and control, representing a significant shift in heuristic and algorithmic approaches.
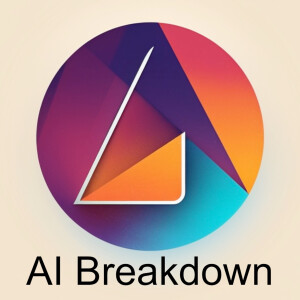
Wednesday Feb 19, 2025
Wednesday Feb 19, 2025
In this episode, we discuss Scaling up Test-Time Compute with Latent Reasoning: A Recurrent Depth Approach by Jonas Geiping, Sean McLeish, Neel Jain, John Kirchenbauer, Siddharth Singh, Brian R. Bartoldson, Bhavya Kailkhura, Abhinav Bhatele, Tom Goldstein. The paper presents a new language model architecture that enhances test-time computation by iteratively reasoning in latent space using a recurrent block, allowing flexible depth during inference. Unlike chain-of-thought approaches, it doesn't require specialized training data, works with small context windows, and can handle complex reasoning not easily expressed in words. A 3.5 billion parameter model was scaled to 800 billion tokens, demonstrating significant performance improvements on reasoning benchmarks with computation loads up to 50 billion parameters.Huggingface: https://huggingface.co/papers/2502.05171
Github: https://github.com/seal-rg/recurrent-pretraining
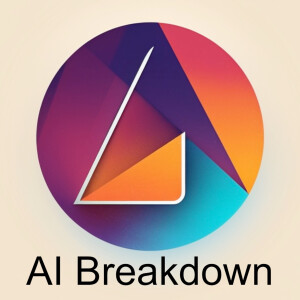
Tuesday Feb 18, 2025
Tuesday Feb 18, 2025
In this episode, we discuss EmbodiedBench: Comprehensive Benchmarking Multi-modal Large Language Models for Vision-Driven Embodied Agents by Rui Yang, Hanyang Chen, Junyu Zhang, Mark Zhao, Cheng Qian, Kangrui Wang, Qineng Wang, Teja Venkat Koripella, Marziyeh Movahedi, Manling Li, Heng Ji, Huan Zhang, Tong Zhang. The paper presents **EMBODIEDBENCH**, a comprehensive benchmark with 1,128 tasks across four environments to evaluate vision-driven embodied agents based on Multi-modal Large Language Models (MLLMs). It assesses key capabilities such as commonsense reasoning, spatial awareness, and long-term planning through six specialized subsets. Evaluations of 13 MLLMs revealed that while these models perform well on high-level tasks, they struggle with low-level manipulations, highlighting significant challenges and guiding future advancements in embodied agent development.
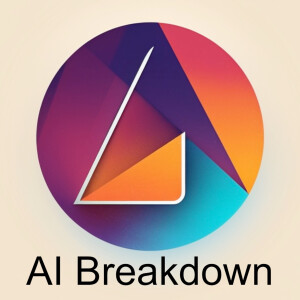
Friday Feb 14, 2025
Friday Feb 14, 2025
In this episode, we discuss VideoEspresso: A Large-Scale Chain-of-Thought Dataset for Fine-Grained Video Reasoning via Core Frame Selection by Songhao Han, Wei Huang, Hairong Shi, Le Zhuo, Xiu Su, Shifeng Zhang, Xu Zhou, Xiaojuan Qi, Yue Liao, Si Liu. The paper introduces VideoEspresso, a high-quality, large-scale VideoQA dataset that maintains essential spatial and temporal details and includes multimodal annotations for intermediate reasoning steps. Utilizing a semantic-aware construction pipeline and GPT-4 for generating QA pairs and Chain-of-Thought annotations, the dataset enhances scalability and reasoning complexity. Additionally, the authors propose a Hybrid LVLMs Collaboration framework that outperforms existing models on 14 tasks, demonstrating superior video reasoning capabilities.
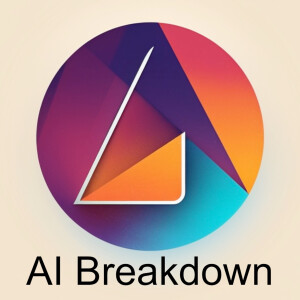
Thursday Feb 13, 2025
Thursday Feb 13, 2025
In this episode, we discuss VideoJAM: Joint Appearance-Motion Representations for Enhanced Motion Generation in Video Models by Hila Chefer, Uriel Singer, Amit Zohar, Yuval Kirstain, Adam Polyak, Yaniv Taigman, Lior Wolf, Shelly Sheynin. Generative video models typically prioritize appearance accuracy over motion coherence, limiting their ability to capture realistic dynamics. The paper presents VideoJAM, a framework that integrates a joint appearance-motion representation and uses an Inner-Guidance mechanism to enhance motion consistency during generation. VideoJAM achieves state-of-the-art motion realism and visual quality while being easily adaptable to existing video models without major changes.
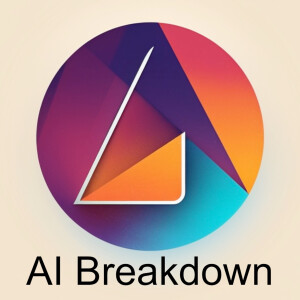
Wednesday Feb 12, 2025
Wednesday Feb 12, 2025
In this episode, we discuss HunyuanVideo: A Systematic Framework For Large Video Generative Models by Weijie Kong, Qi Tian, Zijian Zhang, Rox Min, Zuozhuo Dai, Jin Zhou, Jiangfeng Xiong, Xin Li, Bo Wu, Jianwei Zhang, Kathrina Wu, Qin Lin, Junkun Yuan, Yanxin Long, Aladdin Wang, Andong Wang, Changlin Li, Duojun Huang, Fang Yang, Hao Tan, Hongmei Wang, Jacob Song, Jiawang Bai, Jianbing Wu, Jinbao Xue, Joey Wang, Kai Wang, Mengyang Liu, Pengyu Li, Shuai Li, Weiyan Wang, Wenqing Yu, Xinchi Deng, Yang Li, Yi Chen, Yutao Cui, Yuanbo Peng, Zhentao Yu, Zhiyu He, Zhiyong Xu, Zixiang Zhou, Zunnan Xu, Yangyu Tao, Qinglin Lu, Songtao Liu, Dax Zhou, Hongfa Wang, Yong Yang, Di Wang, Yuhong Liu, Jie Jiang, Caesar Zhong. HunyuanVideo is an innovative open-source video generation model that matches or exceeds the performance of leading closed-source alternatives. It leverages a comprehensive framework encompassing data curation, advanced architecture, progressive scaling, and efficient infrastructure to train a 13-billion-parameter model, the largest of its kind in the open-source domain. Extensive evaluations reveal that HunyuanVideo delivers superior visual quality, motion dynamics, and text-video alignment, and its publicly available code aims to bridge the gap between closed and open-source communities, fostering a more dynamic video generation ecosystem.
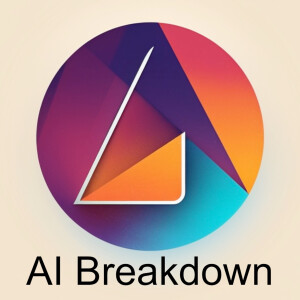
Monday Feb 10, 2025
Monday Feb 10, 2025
In this episode, we discuss s1: Simple test-time scaling by Niklas Muennighoff, Zitong Yang, Weijia Shi, Xiang Lisa Li, Li Fei-Fei, Hannaneh Hajishirzi, Luke Zettlemoyer, Percy Liang, Emmanuel Candès, Tatsunori Hashimoto. The paper introduces a straightforward method for test-time scaling in language models to enhance reasoning performance by utilizing additional computational resources during inference. The authors develop a curated dataset of 1,000 high-quality, diverse, and challenging questions with reasoning traces and implement a "budget forcing" technique that controls the model's computation by either terminating its reasoning process or extending it to encourage double-checking answers. Using this approach, their fine-tuned Qwen2.5-32B-Instruct model outperforms OpenAI’s o1 model on competitive math benchmarks by up to 27% and the resources are made available as open-source.
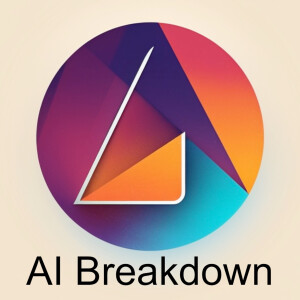
Friday Feb 07, 2025
Friday Feb 07, 2025
In this episode, we discuss Hunyuan3D 2.0: Scaling Diffusion Models for High Resolution Textured 3D Assets Generation by The authors of the paper are the **Hunyuan3D Team**. Specific contributor names are indicated to be listed at the end of the full report.. Hunyuan3D 2.0 is a large-scale 3D synthesis system featuring Hunyuan3D-DiT for generating detailed geometry and Hunyuan3D-Paint for producing high-resolution textures. It includes Hunyuan3D-Studio, a user-friendly platform that allows both professionals and amateurs to efficiently create and manipulate 3D assets. The system outperforms previous models in geometry detail, texture quality, and condition alignment, and it is publicly released to support the open-source 3D community.
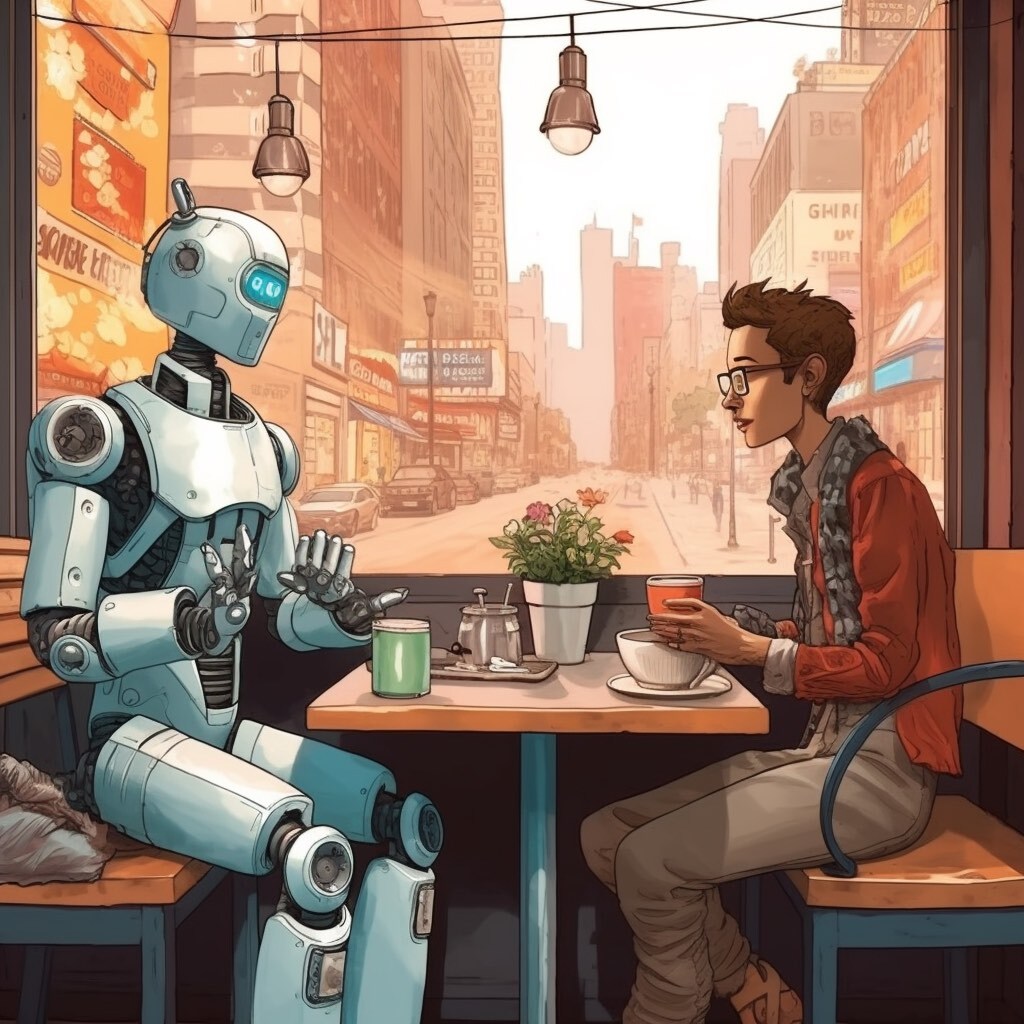
Leverage AI to learn AI
Welcome to the AI Breakdown podcast, where we leverage the power of artificial intelligence to break down recent AI papers and provide simplified explanations of intricate AI topics for educational purposes. We're delighted to have you join us on this exciting journey into the world of artificial intelligence. Our goal is to make complex AI concepts accessible to everyone, and we achieve this by utilizing advanced AI technologies.
Hosts and Ownership: AI Breakdown is under the ownership and management of Megan Maghami and Ramin (Ray) Mehran. Although Megan and Ray lend their voices to the podcast, the content and audio are produced through automated means. Prior to publication, they carefully review the episodes created by AI. They leverage advanced AI technologies, including cutting-edge Large Language Models (LLM) and Text-to-Speech (TTS) systems, to generate captivating episodes. By harnessing these ingenious tools, they deliver enlightening explanations and in-depth analyses on various AI subjects.
Enhancing Your Learning Experience: Your feedback and engagement are crucial to us as we strive to enhance the podcast and provide you with the best possible learning experience. We encourage you to share your thoughts, suggestions, and questions related to our episodes. Together, we can build a vibrant community of AI enthusiasts, learners, and experts, fostering collaboration and knowledge sharing.
Technical Details and Episode Archives: For those interested in the technical aspects behind our AI-generated content, we will provide further insights in upcoming blog posts. Additionally, we will regularly update the blog with published episodes of the AI Breakdown podcast, ensuring convenient access to all our educational resources.