AI Breakdown
The podcast where we use AI to breakdown the recent AI papers and provide simplified explanations of intricate AI topics for educational purposes. The content presented here is generated automatically by utilizing LLM and text to speech technologies. While every effort is made to ensure accuracy, any potential misrepresentations or inaccuracies are unintentional due to evolving technology. We value your feedback to enhance our podcast and provide you with the best possible learning experience.
Episodes
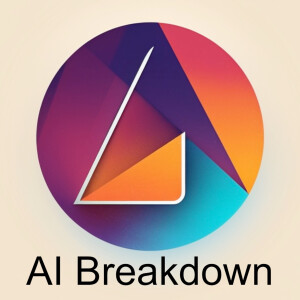
Friday Sep 08, 2023
Friday Sep 08, 2023
In this episode we discuss FACET: Fairness in Computer Vision Evaluation Benchmark
by Laura Gustafson, Chloe Rolland, Nikhila Ravi, Quentin Duval, Aaron Adcock, Cheng-Yang Fu, Melissa Hall, Candace Ross. The paper introduces a new benchmark called FACET, which measures performance disparities in computer vision models across different attributes such as gender and skin tone. It consists of a large evaluation set of 32k images and expert reviewers manually annotated person-related attributes. The benchmark revealed performance disparities across demographic attributes and aims to contribute to the development of fairer and more robust vision models.
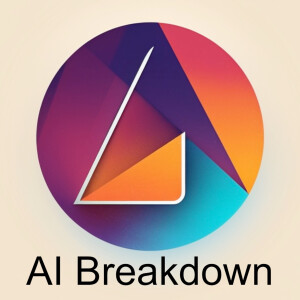
Thursday Sep 07, 2023
Thursday Sep 07, 2023
In this episode we discuss Baseline Defenses for Adversarial Attacks Against Aligned Language Models
by Neel Jain, Avi Schwarzschild, Yuxin Wen, Gowthami Somepalli, John Kirchenbauer, Ping-yeh Chiang, Micah Goldblum, Aniruddha Saha, Jonas Geiping, Tom Goldstein. The paper discusses the security vulnerabilities of Large Language Models (LLMs) and explores defense strategies against adversarial attacks. Three types of defenses are considered: detection, input preprocessing, and adversarial training. The study emphasizes the effectiveness of filtering and preprocessing in LLM defenses and highlights the need for further understanding of LLM security as these models become more prevalent.
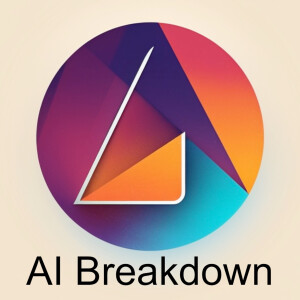
Wednesday Sep 06, 2023
Wednesday Sep 06, 2023
In this episode we discuss Verbs in Action: Improving verb understanding in video-language models
by Liliane Momeni, Mathilde Caron, Arsha Nagrani, Andrew Zisserman, Cordelia Schmid. The paper proposes a Verb-Focused Contrastive (VFC) framework to address the limited understanding of verbs in video-language models. The framework utilizes pre-trained large language models (LLMs) to generate hard negative captions by changing only the verb while keeping the context intact. The method achieves state-of-the-art results in zero-shot performance on three downstream tasks: video-text matching, video question-answering, and video classification.
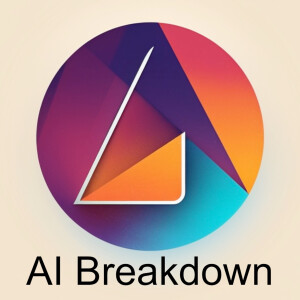
Tuesday Sep 05, 2023
Tuesday Sep 05, 2023
In this episode we discuss RLAIF: Scaling Reinforcement Learning from Human Feedback with AI Feedback
by Harrison Lee, Samrat Phatale, Hassan Mansoor, Kellie Lu, Thomas Mesnard, Colton Bishop, Victor Carbune, Abhinav Rastogi. The paper introduces a new technique called RL from AI Feedback (RLAIF) as a solution to the scalability limitations of reinforcement learning from human feedback (RLHF). RLAIF involves using a large language model (LLM) to label preferences instead of relying on humans. The study compared RLAIF and RLHF on the task of summarization and found that both techniques resulted in similar improvements over a baseline model. Human evaluators preferred both RLAIF and RLHF summaries over the baseline model, suggesting that RLAIF can achieve human-level performance while overcoming the scalability limitations of RLHF.
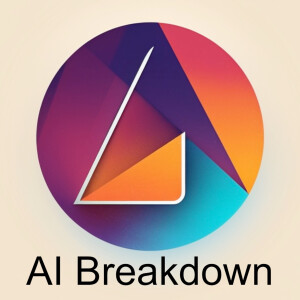
Monday Sep 04, 2023
Monday Sep 04, 2023
In this episode we discuss LLM-Rec: Personalized Recommendation via Prompting Large Language Models
by Hanjia Lyu, Song Jiang, Hanqing Zeng, Qifan Wang, Si Zhang, Ren Chen, Chris Leung, Jiajie Tang, Yinglong Xia, Jiebo Luo. The paper examines different prompting strategies for improving personalized recommendation performance using large language models (LLMs) through input augmentation. The proposed approach, LLM-Rec, incorporates four prompting strategies, demonstrating that using LLM-generated augmented input text enhances recommendation performance. The recommendation-driven and engagement-guided prompting strategies highlight the LLM's comprehension of global and local item characteristics, underscoring the necessity of diverse prompts and input augmentation techniques to enhance recommendation capabilities with LLMs.
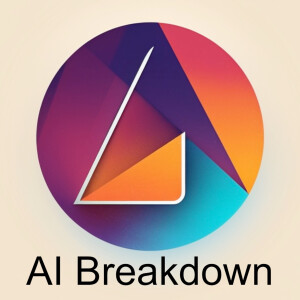
Sunday Sep 03, 2023
Sunday Sep 03, 2023
In this episode we discuss Robust Monocular Depth Estimation under Challenging Conditions
by Stefano Gasperini, Nils Morbitzer, HyunJun Jung, Nassir Navab, Federico Tombari. The paper addresses the limitations of existing monocular depth estimation methods in challenging lighting and weather conditions. The authors propose md4all, a simple and reliable solution that can handle diverse conditions without modification at inference time. The approach involves generating complex training samples, training the model using self- or full-supervision, and computing standard losses on the original images. Extensive experiments on public datasets demonstrate the effectiveness of the approach, surpassing previous works in both standard and challenging conditions.
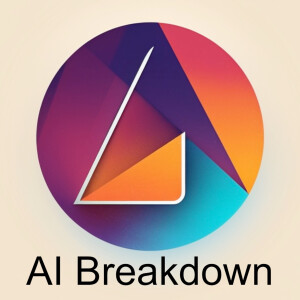
Saturday Sep 02, 2023
Saturday Sep 02, 2023
In this episode we discuss LM-Infinite: Simple On-the-Fly Length Generalization for Large Language Models
by Chi Han, Qifan Wang, Wenhan Xiong, Yu Chen, Heng Ji, Sinong Wang. The paper introduces LM-Infinite as a solution to the challenge of length generalization in Large Language Models (LLMs). Existing methods for handling longer sequences are resource-intensive and time-consuming, prompting the need for a simpler approach. LM-Infinite employs a Λ-shaped attention mask and a distance limit, requiring no parameter updates or learning. The technique consistently generates fluent texts and performs well on downstream tasks even with input sequences exceeding training lengths.
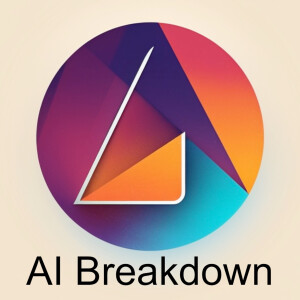
Friday Sep 01, 2023
Friday Sep 01, 2023
In this episode we discuss Llama 2: Open Foundation and Fine-Tuned Chat Models
by Hugo Touvron, Louis Martin, Kevin Stone, Peter Albert, Amjad Almahairi, Yasmine Babaei, Nikolay Bashlykov, Soumya Batra, Prajjwal Bhargava, Shruti Bhosale, Dan Bikel, Lukas Blecher, Cristian Canton Ferrer, Moya Chen, Guillem Cucurull, David Esiobu, Jude Fernandes, Jeremy Fu, Wenyin Fu, Brian Fuller, Cynthia Gao, Vedanuj Goswami, Naman Goyal, Anthony Hartshorn, Saghar Hosseini, Rui Hou, Hakan Inan, Marcin Kardas, Viktor Kerkez, Madian Khabsa, Isabel Kloumann, Artem Korenev, Punit Singh Koura, Marie-Anne Lachaux, Thibaut Lavril, Jenya Lee, Diana Liskovich, Yinghai Lu, Yuning Mao, Xavier Martinet, Todor Mihaylov, Pushkar Mishra, Igor Molybog, Yixin Nie, Andrew Poulton, Jeremy Reizenstein, Rashi Rungta, Kalyan Saladi, Alan Schelten, Ruan Silva, Eric Michael Smith, Ranjan Subramanian, Xiaoqing Ellen Tan, Binh Tang, Ross Taylor, Adina Williams, Jian Xiang Kuan, Puxin Xu, Zheng Yan, Iliyan Zarov, Yuchen Zhang, Angela Fan, Melanie Kambadur, Sharan Narang, Aurelien Rodriguez, Robert Stojnic, Sergey Edunov, Thomas Scialom. The paper introduces Llama 2, a collection of pretrained and fine-tuned large language models optimized for dialogue purposes. Ranging from 7 billion to 70 billion parameters, the Llama 2-Chat models surpass existing open-source chat models in performance across different benchmarks. The authors also conduct human evaluations, indicating that their models could be viable alternatives to closed-source models, and provide detailed insights into their fine-tuning process and safety enhancements.
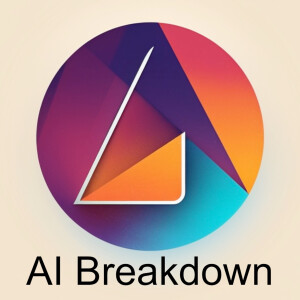
Thursday Aug 31, 2023
Thursday Aug 31, 2023
In this episode we discuss Nougat: Neural Optical Understanding for Academic Documents
by Lukas Blecher, Guillem Cucurull, Thomas Scialom, Robert Stojnic. The paper introduces Nougat, a neural optical understanding model for academic documents. Nougat utilizes a Visual Transformer model and Optical Character Recognition (OCR) to convert scientific documents into a markup language, bridging the gap between human-readable and machine-readable text. The method is versatile, capable of processing scanned papers and books, and includes a pre-trained model and code on GitHub, as well as a pipeline for creating datasets.
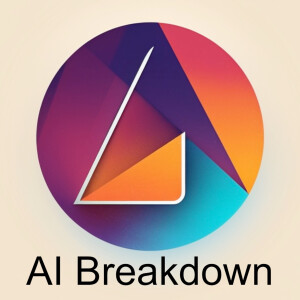
Wednesday Aug 30, 2023
Wednesday Aug 30, 2023
In this episode we discuss Graph of Thoughts: Solving Elaborate Problems with Large Language Models
by Maciej Besta, Nils Blach, Ales Kubicek, Robert Gerstenberger, Lukas Gianinazzi, Joanna Gajda, Tomasz Lehmann, Michal Podstawski, Hubert Niewiadomski, Piotr Nyczyk, Torsten Hoefler. The paper introduces a framework called Graph of Thoughts (GoT) that enhances the prompting capabilities of large language models (LLMs). GoT models the information generated by an LLM as an arbitrary graph, where LLM thoughts are vertices and edges represent dependencies between these thoughts. The paper demonstrates that GoT outperforms state-of-the-art methods on different tasks and can be used to spearhead new prompting schemes.
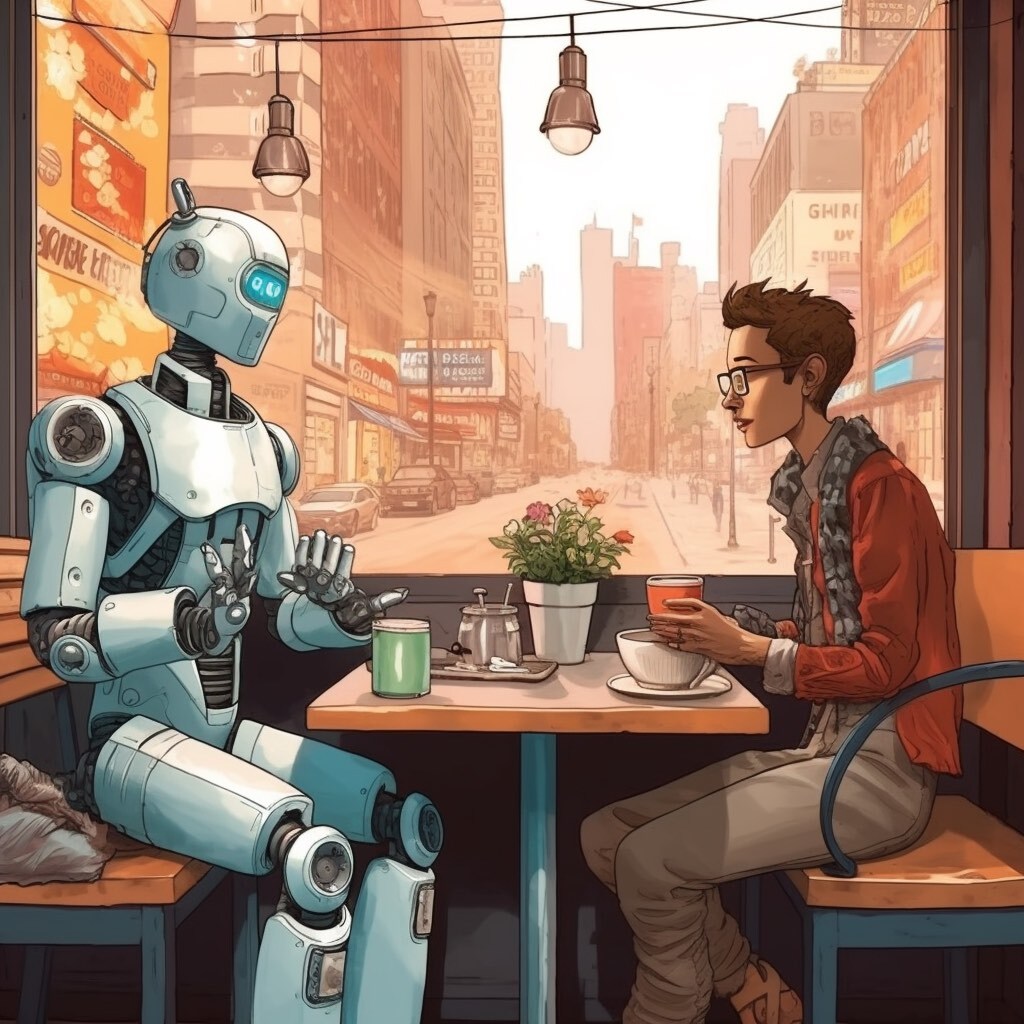
Leverage AI to learn AI
Welcome to the AI Breakdown podcast, where we leverage the power of artificial intelligence to break down recent AI papers and provide simplified explanations of intricate AI topics for educational purposes. We're delighted to have you join us on this exciting journey into the world of artificial intelligence. Our goal is to make complex AI concepts accessible to everyone, and we achieve this by utilizing advanced AI technologies.
Hosts and Ownership: AI Breakdown is under the ownership and management of Megan Maghami and Ramin (Ray) Mehran. Although Megan and Ray lend their voices to the podcast, the content and audio are produced through automated means. Prior to publication, they carefully review the episodes created by AI. They leverage advanced AI technologies, including cutting-edge Large Language Models (LLM) and Text-to-Speech (TTS) systems, to generate captivating episodes. By harnessing these ingenious tools, they deliver enlightening explanations and in-depth analyses on various AI subjects.
Enhancing Your Learning Experience: Your feedback and engagement are crucial to us as we strive to enhance the podcast and provide you with the best possible learning experience. We encourage you to share your thoughts, suggestions, and questions related to our episodes. Together, we can build a vibrant community of AI enthusiasts, learners, and experts, fostering collaboration and knowledge sharing.
Technical Details and Episode Archives: For those interested in the technical aspects behind our AI-generated content, we will provide further insights in upcoming blog posts. Additionally, we will regularly update the blog with published episodes of the AI Breakdown podcast, ensuring convenient access to all our educational resources.