AI Breakdown
The podcast where we use AI to breakdown the recent AI papers and provide simplified explanations of intricate AI topics for educational purposes. The content presented here is generated automatically by utilizing LLM and text to speech technologies. While every effort is made to ensure accuracy, any potential misrepresentations or inaccuracies are unintentional due to evolving technology. We value your feedback to enhance our podcast and provide you with the best possible learning experience.
Episodes
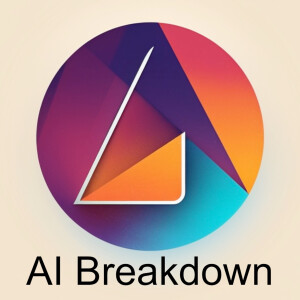
Friday Mar 28, 2025
Friday Mar 28, 2025
In this episode, we discuss SynCity: Training-Free Generation of 3D Worlds by Paul Engstler, Aleksandar Shtedritski, Iro Laina, Christian Rupprecht, Andrea Vedaldi. The paper presents SynCity, a novel method for generating expansive 3D worlds directly from textual descriptions without requiring additional training or optimization. SynCity combines the geometric accuracy of pre-trained 3D generative models with the creative flexibility of 2D image generators using a tile-based approach, enabling detailed and controlled scene layouts. This tile-by-tile generation and seamless fusion process results in large, high-quality, and immersive 3D environments rich in detail and diversity.
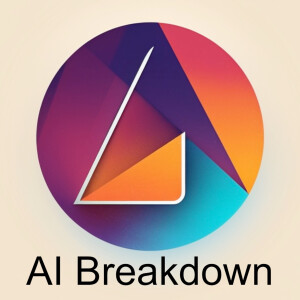
Wednesday Mar 26, 2025
Wednesday Mar 26, 2025
In this episode, we discuss HD-EPIC: A Highly-Detailed Egocentric Video Dataset by Toby Perrett, Ahmad Darkhalil, Saptarshi Sinha, Omar Emara, Sam Pollard, Kranti Parida, Kaiting Liu, Prajwal Gatti, Siddhant Bansal, Kevin Flanagan, Jacob Chalk, Zhifan Zhu, Rhodri Guerrier, Fahd Abdelazim, Bin Zhu, Davide Moltisanti, Michael Wray, Hazel Doughty, Dima Damen. The paper introduces HD-EPIC, a 41-hour dataset of egocentric kitchen videos collected from diverse home environments and meticulously annotated with detailed 3D-grounded labels, including recipe steps, actions, ingredients, and audio events. It features a challenging visual question answering benchmark with 26,000 questions, where current models like Gemini Pro achieve only 38.5% accuracy, underscoring the dataset's complexity and the limitations of existing vision-language models. Additionally, HD-EPIC supports various tasks such as action recognition and video-object segmentation, providing a valuable resource for enhancing real-world kitchen scenario understanding.
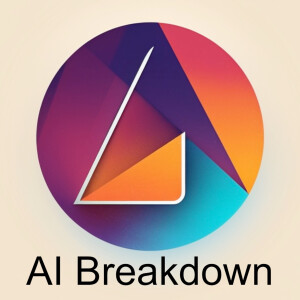
Tuesday Mar 25, 2025
Tuesday Mar 25, 2025
In this episode, we discuss Video-T1: Test-Time Scaling for Video Generation by Fangfu Liu, Hanyang Wang, Yimo Cai, Kaiyan Zhang, Xiaohang Zhan, Yueqi Duan. The paper investigates Test-Time Scaling (TTS) for video generation, aiming to enhance video quality by leveraging additional inference-time computation instead of expanding model size or training data. The authors treat video generation as a search problem, introducing the Tree-of-Frames (ToF) method, which efficiently navigates the search space by adaptively expanding and pruning video branches based on feedback from test-time verifiers. Experimental results on text-conditioned video benchmarks show that increasing inference-time compute through TTS significantly improves the quality of the generated videos.
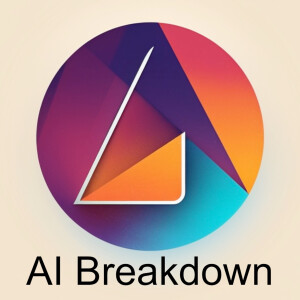
Monday Mar 24, 2025
Monday Mar 24, 2025
In this episode, we discuss Calibrated Multi-Preference Optimization for Aligning Diffusion Models by Kyungmin Lee, Xiaohang Li, Qifei Wang, Junfeng He, Junjie Ke, Ming-Hsuan Yang, Irfan Essa, Jinwoo Shin, Feng Yang, Yinxiao Li. The paper introduces Calibrated Preference Optimization (CaPO), a new method for aligning text-to-image diffusion models using multiple reward models without requiring expensive human-annotated data. CaPO calibrates general preferences by calculating expected win-rates against pretrained model samples and employs a frontier-based pair selection to handle multi-preference distributions effectively. Experimental evaluations on benchmarks like GenEval and T2I-Compbench show that CaPO consistently outperforms existing methods such as Direct Preference Optimization in both single and multi-reward scenarios.
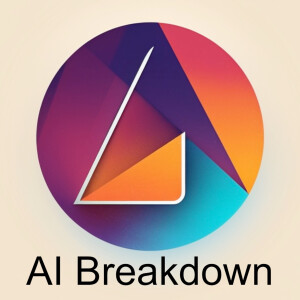
Friday Mar 21, 2025
Friday Mar 21, 2025
In this episode, we discuss Personalize Anything for Free with Diffusion Transformer by Haoran Feng, Zehuan Huang, Lin Li, Hairong Lv, Lu Sheng. The paper introduces *Personalize Anything*, a training-free framework for personalized image generation using diffusion transformers (DiTs). By replacing denoising tokens with those of a reference subject, the method enables zero-shot subject reconstruction and supports flexible editing scenarios. Evaluations show that this approach achieves state-of-the-art performance in identity preservation and versatility, offering efficient personalization without the need for training.
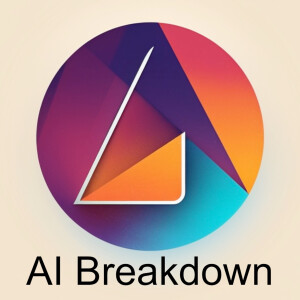
Thursday Mar 20, 2025
Thursday Mar 20, 2025
In this episode, we discuss Story-Adapter: A Training-free Iterative Framework for Long Story Visualization by Jiawei Mao, Xiaoke Huang, Yunfei Xie, Yuanqi Chang, Mude Hui, Bingjie Xu, Yuyin Zhou. The paper tackles the challenge of generating coherent image sequences for long narratives using text-to-image diffusion models. It introduces Story-Adapter, a training-free and efficient framework that iteratively refines each image by incorporating the text prompt and previously generated images. This method enhances semantic consistency and detail quality across up to 100 frames without the need for additional training.
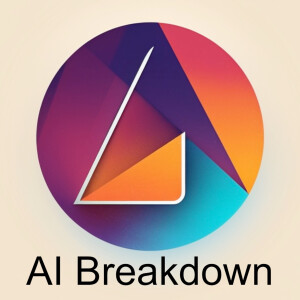
Tuesday Mar 18, 2025
Tuesday Mar 18, 2025
In this episode, we discuss ReCamMaster: Camera-Controlled Generative Rendering from A Single Video by Jianhong Bai, Menghan Xia, Xiao Fu, Xintao Wang, Lianrui Mu, Jinwen Cao, Zuozhu Liu, Haoji Hu, Xiang Bai, Pengfei Wan, Di Zhang. ReCamMaster is a generative framework that modifies camera trajectories in existing videos by re-rendering scenes from new perspectives. It utilizes pre-trained text-to-video models with a unique video conditioning mechanism and is trained on a diverse, multi-camera dataset created using Unreal Engine 5 to ensure real-world applicability. Comprehensive experiments demonstrate that ReCamMaster outperforms current state-of-the-art methods and is effective in applications like video stabilization, super-resolution, and outpainting.
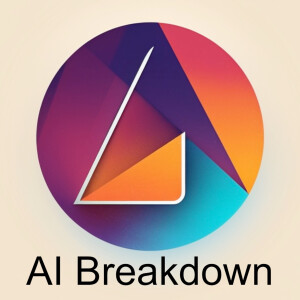
Monday Mar 17, 2025
Monday Mar 17, 2025
In this episode, we discuss Vision-R1: Incentivizing Reasoning Capability in Multimodal Large Language Models by Wenxuan Huang, Bohan Jia, Zijie Zhai, Shaosheng Cao, Zheyu Ye, Fei Zhao, Zhe Xu, Yao Hu, Shaohui Lin. The paper aims to enhance the reasoning abilities of Multimodal Large Language Models (MLLMs) using reinforcement learning (RL). To overcome the lack of high-quality multimodal reasoning data, the authors develop Vision-R1 by creating a 200K multimodal Chain-of-Thought dataset without human annotations. They further improve Vision-R1’s reasoning through Progressive Thinking Suppression Training and Group Relative Policy Optimization on a specialized 10K multimodal math dataset.
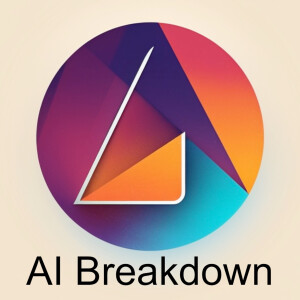
Thursday Mar 13, 2025
Thursday Mar 13, 2025
In this episode, we discuss MEGA-Bench: Scaling Multimodal Evaluation to over 500 Real-World Tasks by Jiacheng Chen, Tianhao Liang, Sherman Siu, Zhengqing Wang, Kai Wang, Yubo Wang, Yuansheng Ni, Wang Zhu, Ziyan Jiang, Bohan Lyu, Dongfu Jiang, Xuan He, Yuan Liu, Hexiang Hu, Xiang Yue, Wenhu Chen. The paper introduces MEGA-BENCH, a comprehensive evaluation suite featuring over 500 real-world multimodal tasks to address diverse daily user needs. It includes more than 8,000 samples curated by 16 expert annotators, utilizing a variety of output formats such as numbers, phrases, and code instead of standard multiple-choice questions. MEGA-BENCH aims to provide high-quality, diverse data for cost-effective and accurate model evaluation across a wide range of multimodal tasks.
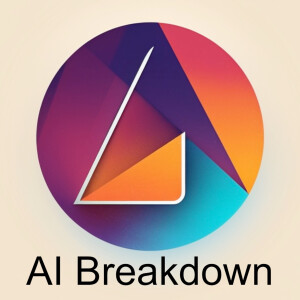
Wednesday Mar 12, 2025
Wednesday Mar 12, 2025
In this episode, we discuss TrajectoryCrafter: Redirecting Camera Trajectory for Monocular Videos via Diffusion Models by Mark YU, Wenbo Hu, Jinbo Xing, Ying Shan. TrajectoryCrafter is a new method that precisely redirects camera paths in monocular videos by separating view changes from content generation. It uses a dual-stream conditional video diffusion model that combines point cloud renders with source videos to ensure accurate views and coherent 4D content. By training on a hybrid dataset of monocular and multi-view videos with a double-reprojection strategy, TrajectoryCrafter achieves robust performance across diverse scenes.
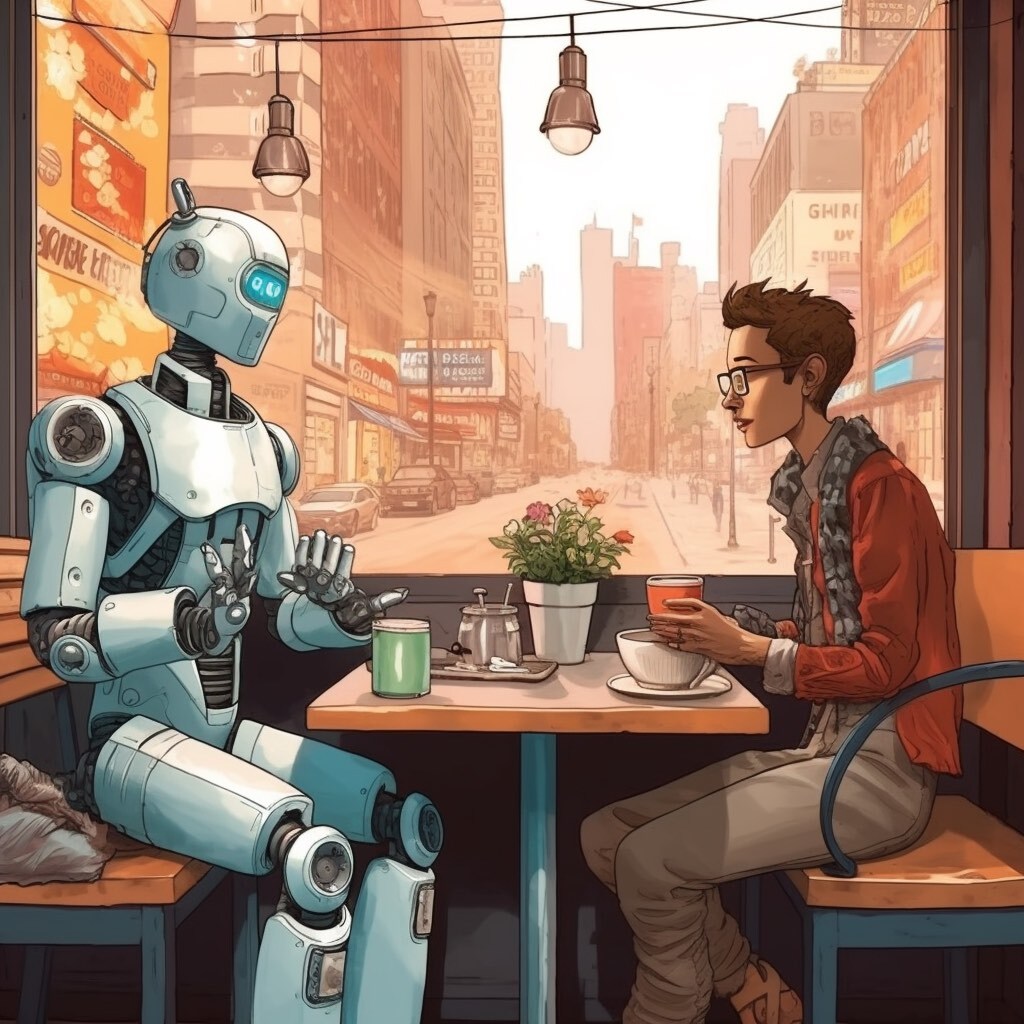
Leverage AI to learn AI
Welcome to the AI Breakdown podcast, where we leverage the power of artificial intelligence to break down recent AI papers and provide simplified explanations of intricate AI topics for educational purposes. We're delighted to have you join us on this exciting journey into the world of artificial intelligence. Our goal is to make complex AI concepts accessible to everyone, and we achieve this by utilizing advanced AI technologies.
Hosts and Ownership: AI Breakdown is under the ownership and management of Megan Maghami and Ramin (Ray) Mehran. Although Megan and Ray lend their voices to the podcast, the content and audio are produced through automated means. Prior to publication, they carefully review the episodes created by AI. They leverage advanced AI technologies, including cutting-edge Large Language Models (LLM) and Text-to-Speech (TTS) systems, to generate captivating episodes. By harnessing these ingenious tools, they deliver enlightening explanations and in-depth analyses on various AI subjects.
Enhancing Your Learning Experience: Your feedback and engagement are crucial to us as we strive to enhance the podcast and provide you with the best possible learning experience. We encourage you to share your thoughts, suggestions, and questions related to our episodes. Together, we can build a vibrant community of AI enthusiasts, learners, and experts, fostering collaboration and knowledge sharing.
Technical Details and Episode Archives: For those interested in the technical aspects behind our AI-generated content, we will provide further insights in upcoming blog posts. Additionally, we will regularly update the blog with published episodes of the AI Breakdown podcast, ensuring convenient access to all our educational resources.