AI Breakdown
The podcast where we use AI to breakdown the recent AI papers and provide simplified explanations of intricate AI topics for educational purposes. The content presented here is generated automatically by utilizing LLM and text to speech technologies. While every effort is made to ensure accuracy, any potential misrepresentations or inaccuracies are unintentional due to evolving technology. We value your feedback to enhance our podcast and provide you with the best possible learning experience.
Episodes
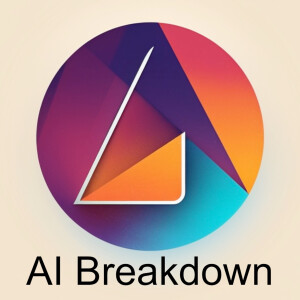
Thursday Jun 13, 2024
Thursday Jun 13, 2024
In this episode, we discuss TextGrad: Automatic "Differentiation" via Text by Mert Yuksekgonul, Federico Bianchi, Joseph Boen, Sheng Liu, Zhi Huang, Carlos Guestrin, James Zou. The paper introduces TEXTGRAD, a novel framework that automates the optimization of compound AI systems by utilizing textual feedback from large language models (LLMs). TEXTGRAD treats text feedback as a form of "differentiation" to improve the components of these AI systems across various applications, working out-of-the-box without requiring specific tuning. Demonstrating its effectiveness, TEXTGRAD enhances performance in diverse tasks such as question answering, coding problem solutions, molecule design, and treatment planning, marking a significant step forward for the development of advanced AI technologies.
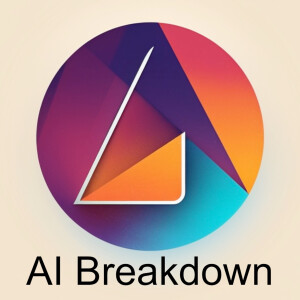
Wednesday Jun 12, 2024
Wednesday Jun 12, 2024
In this episode, we discuss SaySelf: Teaching LLMs to Express Confidence with Self-Reflective Rationales by Tianyang Xu, Shujin Wu, Shizhe Diao, Xiaoze Liu, Xingyao Wang, Yangyi Chen, Jing Gao. The paper introduces SaySelf, a framework for training large language models (LLMs) to produce accurate, fine-grained confidence estimates and self-reflective rationales explaining their uncertainties. This is achieved by analyzing inconsistencies in multiple reasoning chains, summarizing uncertainties in natural language, and applying supervised fine-tuning alongside reinforcement learning to calibrate confidence levels. Experimental results show that SaySelf effectively reduces confidence calibration errors and maintains task performance, enhancing LLMs' reliability by mitigating overconfidence in erroneous outputs.
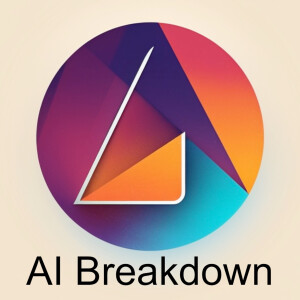
Tuesday Jun 11, 2024
Tuesday Jun 11, 2024
In this episode, we discuss Open-Endedness is Essential for Artificial Superhuman Intelligence by Edward Hughes, Michael Dennis, Jack Parker-Holder, Feryal Behbahani, Aditi Mavalankar, Yuge Shi, Tom Schaul, Tim Rocktaschel. The paper argues that the development of open-ended, self-improving AI systems is achievable using current foundation models trained on extensive internet data. It provides a formal definition of open-endedness based on novelty and learnability and suggests a path to artificial superhuman intelligence (ASI) through such systems. The paper emphasizes the importance of considering safety in the development of these highly capable and open-ended AI systems.
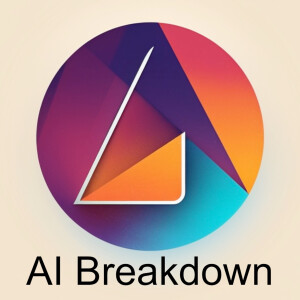
Friday Jun 07, 2024
Friday Jun 07, 2024
In this episode, we discuss To Believe or Not to Believe Your LLM by Yasin Abbasi Yadkori, Ilja Kuzborskij, András György, Csaba Szepesvári. The study investigates uncertainty quantification in large language models (LLMs), focusing on distinguishing large epistemic uncertainty to identify unreliable outputs and potential hallucinations. By employing an information-theoretic metric and a method of iterative prompting based on prior responses, the approach effectively detects high uncertainty scenarios, particularly in distinguishing between cases with single and multiple possible answers. The proposed method outperforms standard strategies and highlights how iterative prompting influences the probability assignments of LLM outputs.
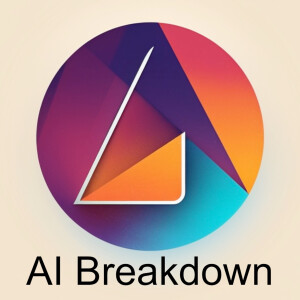
Wednesday Jun 05, 2024
Wednesday Jun 05, 2024
In this episode, we discuss Similarity is Not All You Need: Endowing Retrieval Augmented Generation with Multi Layered Thoughts by Chunjing Gan, Dan Yang, Binbin Hu, Hanxiao Zhang, Siyuan Li, Ziqi Liu, Yue Shen, Lin Ju, Zhiqiang Zhang, Jinjie Gu, Lei Liang, Jun Zhou. The paper introduces METRAG, a novel Multi-layered Thought enhanced Retrieval-Augmented Generation framework designed to improve the performance of LLMs in knowledge-intensive tasks. Unlike traditional models that solely rely on similarity for document retrieval, METRAG combines similarity-oriented, utility-oriented, and compactness-oriented thoughts to enhance the retrieval and generation process. The framework has shown superior results in various experiments, addressing concerns about knowledge update delays, cost, and hallucinations in LLMs.
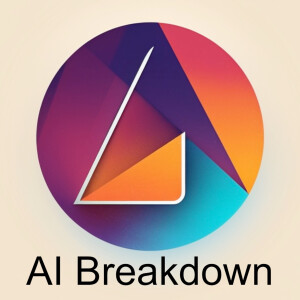
Tuesday Jun 04, 2024
Tuesday Jun 04, 2024
In this episode, we discuss Contextual Position Encoding: Learning to Count What's Important by Olga Golovneva, Tianlu Wang, Jason Weston, Sainbayar Sukhbaatar. The paper introduces Contextual Position Encoding (CoPE), a new position encoding method for Large Language Models (LLMs) that incrementally alters position based on context rather than just token count. This approach enables more sophisticated addressing, such as targeting specific types of words or sentences, beyond the capabilities of current token-based methods. Through experiments, CoPE demonstrates improved performance on tasks like selective copy, counting, and Flip-Flop, as well as enhancements in language modeling and coding task perplexity.
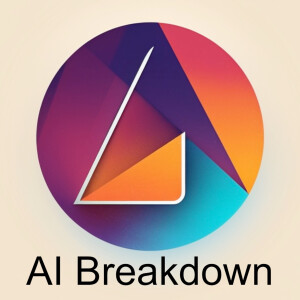
Monday Jun 03, 2024
Monday Jun 03, 2024
In this episode, we discuss Video-MME: The First-Ever Comprehensive Evaluation Benchmark of Multi-modal LLMs in Video Analysis by Chaoyou Fu, Yuhan Dai, Yondong Luo, Lei Li, Shuhuai Ren, Renrui Zhang, Zihan Wang, Chenyu Zhou, Yunhang Shen, Mengdan Zhang, Peixian Chen, Yanwei Li, Shaohui Lin, Sirui Zhao, Ke Li, Tong Xu, Xiawu Zheng, Enhong Chen, Rongrong Ji, Xing Sun. The paper introduces Video-MME, a comprehensive benchmark for evaluating Multi-modal Large Language Models (MLLMs) in video analysis, which assesses capabilities across diverse video types, durations, and data modalities with high-quality annotations. Their experiments show commercial models like Gemini 1.5 Pro outperform open-source counterparts and highlight the significant impact of subtitles and audio on video understanding, along with a noted drop in model performance with longer videos. The findings emphasize the need for improvements in handling extended sequences and multi-modal data, driving future advancements in MLLM capabilities.
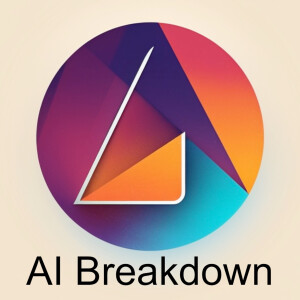
Friday May 31, 2024
Friday May 31, 2024
In this episode, we discuss VideoTree: Adaptive Tree-based Video Representation for LLM Reasoning on Long Videos by Ziyang Wang, Shoubin Yu, Elias Stengel-Eskin, Jaehong Yoon, Feng Cheng, Gedas Bertasius, Mohit Bansal. The paper introduces VideoTree, a novel framework that enhances the efficiency and accuracy of long-video question answering by selectively extracting and hierarchically organizing frames based on their relevance to the query. Unlike traditional methods that rely on dense and often redundant sampling of frames for LLM-based reasoning, VideoTree employs a dynamic, adaptive approach to identify and caption keyframes, forming a tree structure that reflects varying levels of detail where needed. Experiments demonstrate significant performance improvements and reduced inference times on benchmarks like EgoSchema, NExT-QA, and IntentQA.
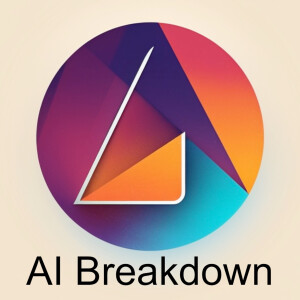
Thursday May 30, 2024
Thursday May 30, 2024
In this episode, we discuss CinePile: A Long Video Question Answering Dataset and Benchmark by Ruchit Rawal, Khalid Saifullah, Ronen Basri, David Jacobs, Gowthami Somepalli, Tom Goldstein. CinePile is a new dataset and benchmark designed for authentic long-form video understanding, addressing the limitations of current datasets. It comprises 305,000 multiple-choice questions (MCQs) spanning various visual and multimodal aspects. The evaluation of recent state-of-the-art video-centric language models (LLMs) shows a significant gap between machine and human performance in these complex tasks.
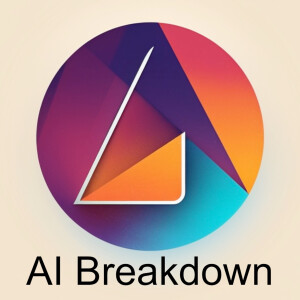
Wednesday May 29, 2024
Wednesday May 29, 2024
In this episode, we discuss Dataset Decomposition: Faster LLM Training with Variable Sequence Length Curriculum by Hadi Pouransari, Chun-Liang Li, Jen-Hao Rick Chang, Pavan Kumar Anasosalu Vasu, Cem Koc, Vaishaal Shankar, Oncel Tuzel. The paper introduces a novel variable sequence length training technique called dataset decomposition to address inefficiencies in training large language models (LLMs) with fixed-length token sequences. It divides the dataset into buckets of sequences of the same size from unique documents and samples from these buckets with a curriculum during training, leading to computational savings and higher efficiency. This approach achieves target accuracy three times faster than traditional methods and enhances performance on standard language evaluations and long-context benchmarks.
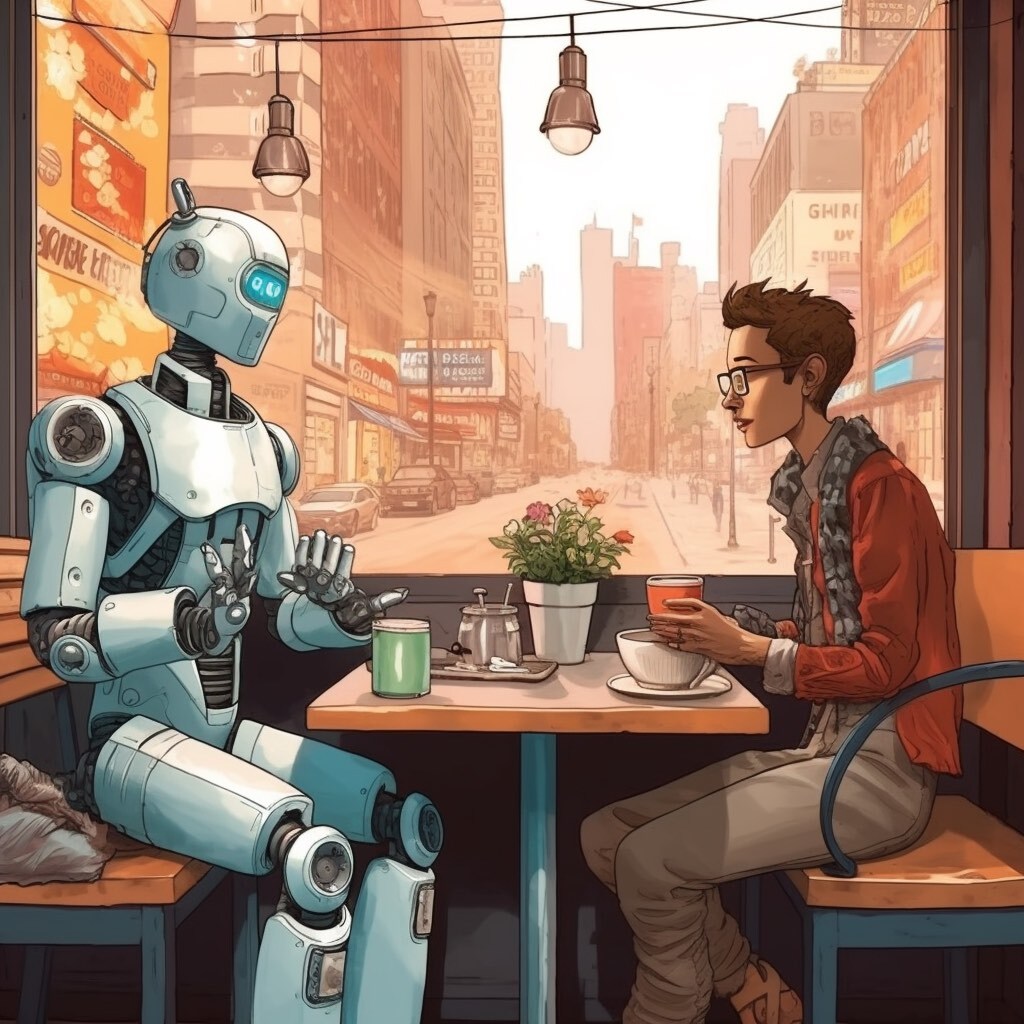
Leverage AI to learn AI
Welcome to the AI Breakdown podcast, where we leverage the power of artificial intelligence to break down recent AI papers and provide simplified explanations of intricate AI topics for educational purposes. We're delighted to have you join us on this exciting journey into the world of artificial intelligence. Our goal is to make complex AI concepts accessible to everyone, and we achieve this by utilizing advanced AI technologies.
Hosts and Ownership: AI Breakdown is under the ownership and management of Megan Maghami and Ramin (Ray) Mehran. Although Megan and Ray lend their voices to the podcast, the content and audio are produced through automated means. Prior to publication, they carefully review the episodes created by AI. They leverage advanced AI technologies, including cutting-edge Large Language Models (LLM) and Text-to-Speech (TTS) systems, to generate captivating episodes. By harnessing these ingenious tools, they deliver enlightening explanations and in-depth analyses on various AI subjects.
Enhancing Your Learning Experience: Your feedback and engagement are crucial to us as we strive to enhance the podcast and provide you with the best possible learning experience. We encourage you to share your thoughts, suggestions, and questions related to our episodes. Together, we can build a vibrant community of AI enthusiasts, learners, and experts, fostering collaboration and knowledge sharing.
Technical Details and Episode Archives: For those interested in the technical aspects behind our AI-generated content, we will provide further insights in upcoming blog posts. Additionally, we will regularly update the blog with published episodes of the AI Breakdown podcast, ensuring convenient access to all our educational resources.