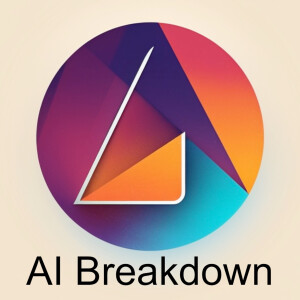
Sunday Jul 23, 2023
ICML 2023 - Self-Repellent Random Walks on General Graphs -- Achieving Minimal Sampling Variance via Nonlinear Markov Chains
In this episode we discuss Self-Repellent Random Walks on General Graphs -- Achieving Minimal Sampling Variance via Nonlinear Markov Chains by Vishwaraj Doshi, Jie Hu, Do Young Eun. This paper introduces self-repellent random walks (SRRWs) as a way to improve sampling efficiency in Markov chain Monte Carlo (MCMC) procedures. It proves that the SRRWs converge to the target distribution, provides a central limit theorem and covariance matrix, and shows that stronger repellence leads to smaller asymptotic covariance. The paper also demonstrates that the decrease in sampling variance for SRRW-driven MCMC algorithms is of the order O(1/α), where α controls the strength of repellence.
Comments (0)
To leave or reply to comments, please download free Podbean or
No Comments
To leave or reply to comments,
please download free Podbean App.