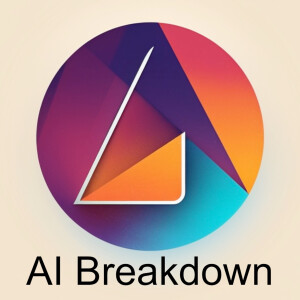
Tuesday Oct 17, 2023
ICCV 2023 - Sigmoid Loss for Language Image Pre-Training
In this episode we discuss Sigmoid Loss for Language Image Pre-Training by Xiaohua Zhai, Basil Mustafa, Alexander Kolesnikov, Lucas Beyer. The paper introduces a pairwise Sigmoid loss for Language-Image Pre-training (SigLIP), which operates on image-text pairs and allows for scaling up batch size without the need for global pairwise similarities. By combining SigLIP with Locked-image Tuning, the authors achieve high ImageNet zero-shot accuracy in just two days of training. The authors also discuss the impact of batch size and find that a batch size of 32k is sufficient.
Comments (0)
To leave or reply to comments, please download free Podbean or
No Comments
To leave or reply to comments,
please download free Podbean App.