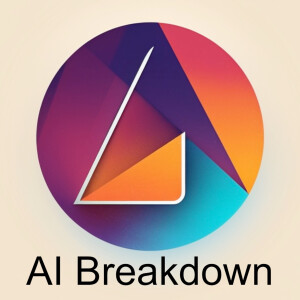
Saturday May 20, 2023
CVPR 2023 - The Resource Problem of Using Linear Layer Leakage Attack in Federated Learning
In this episode we discuss The Resource Problem of Using Linear Layer Leakage Attack in Federated Learning by Joshua C. Zhao, Ahmed Roushdy Elkordy, Atul Sharma, Yahya H. Ezzeldin, Salman Avestimehr, Saurabh Bagchi. The paper discusses the usage of secure aggregation in federated learning, which promises to maintain privacy by only allowing the server access to a decrypted aggregate update. The paper focuses on linear layer leakage methods, which are the only data reconstruction attacks that can scale regardless of the number of clients or batch sizes. However, the method of injecting a fully-connected layer to increase the leakage rate results in a large resource overhead. The authors propose using sparsity to decrease the model size overhead and computation time while maintaining an equivalent total leakage rate of 77%.
No comments yet. Be the first to say something!