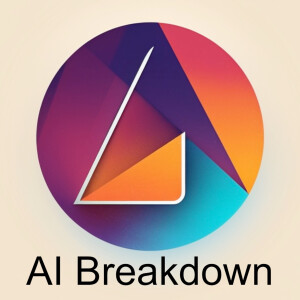
Thursday May 25, 2023
CVPR 2023 - Progressive Random Convolutions for Single Domain Generalization
In this episode we discuss Progressive Random Convolutions for Single Domain Generalization by Seokeon Choi, Debasmit Das, Sungha Choi, Seunghan Yang, Hyunsin Park, Sungrack Yun. The paper proposes a method called Progressive Random Convolution (Pro-RandConv) for single domain generalization, which aims to train a model with only one source domain to perform well on arbitrary unseen target domains. The proposed method recursively stacks random convolution layers with a small kernel size instead of increasing the kernel size, which can mitigate semantic distortions and create more effective virtual domains. They also develop a random convolution block to support texture and contrast diversification. Without complex generators or adversarial learning, the proposed method outperforms state-of-the-art methods on single domain generalization benchmarks.
Comments (0)
To leave or reply to comments, please download free Podbean or
No Comments
To leave or reply to comments,
please download free Podbean App.