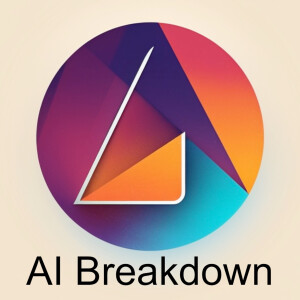
Sunday May 21, 2023
CVPR 2023 - FJMP: Factorized Joint Multi-Agent Motion Prediction over Learned Directed Acyclic Interaction Graphs
In this episode we discuss FJMP: Factorized Joint Multi-Agent Motion Prediction over Learned Directed Acyclic Interaction Graphs by Luke Rowe, Martin Ethier, Eli-Henry Dykhne, Krzysztof Czarnecki. The paper proposes a framework called FJMP for generating a set of joint future trajectory predictions in multi-agent driving scenarios. FJMP models the future scene interaction dynamics using a sparse directed interaction graph and decomposes the joint prediction task into a sequence of marginal and conditional predictions according to the partial ordering of the graph. The results show that FJMP outperforms non-factorized approaches and ranks 1st on the multi-agent test leaderboard of the INTERACTION dataset.
No comments yet. Be the first to say something!