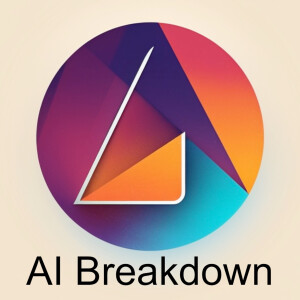
Friday May 19, 2023
CVPR 2023 - ANetQA: A Large-scale Benchmark for Fine-grained Compositional Reasoning over Untrimmed Videos
In this episode we discuss ANetQA: A Large-scale Benchmark for Fine-grained Compositional Reasoning over Untrimmed Videos by Zhou Yu, Lixiang Zheng, Zhou Zhao, Fei Wu, Jianping Fan, Kui Ren, Jun Yu. The paper discusses the challenge of building benchmarks for video question answering (VideoQA) models that can systematically analyze their capabilities. Existing benchmarks have limitations such as non-compositional simple questions and language biases. The authors present ANetQA, a large-scale benchmark that supports fine-grained compositional reasoning on untrimmed videos from ActivityNet, with spatio-temporal scene graphs and diverse questions generated from fine-grained templates. The benchmark attains 1.4 billion unbalanced and 13.4 million balanced QA pairs, and comprehensive experiments are performed for state-of-the-art methods, with the best model achieving 44.5% accuracy and human performance topping out at 84.5%.
Comments (0)
To leave or reply to comments, please download free Podbean or
No Comments
To leave or reply to comments,
please download free Podbean App.