AI Breakdown
The podcast where we use AI to breakdown the recent AI papers and provide simplified explanations of intricate AI topics for educational purposes. The content presented here is generated automatically by utilizing LLM and text to speech technologies. While every effort is made to ensure accuracy, any potential misrepresentations or inaccuracies are unintentional due to evolving technology. We value your feedback to enhance our podcast and provide you with the best possible learning experience.
Episodes
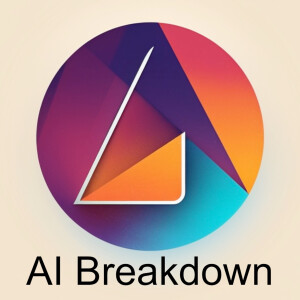
22 hours ago
22 hours ago
In this episode, we discuss F5-TTS: A Fairytaler that Fakes Fluent and Faithful Speech with Flow Matching by Yushen Chen, Zhikang Niu, Ziyang Ma, Keqi Deng, Chunhui Wang, Jian Zhao, Kai Yu, Xie Chen. F5-TTS is a fully non-autoregressive text-to-speech system that utilizes flow matching with Diffusion Transformer (DiT) and addresses limitations of previous systems like E2 TTS by padding text inputs with filler tokens to match speech input lengths. It includes ConvNeXt for refining text representations and employs a new Sway Sampling strategy to enhance performance during inference without retraining. The system achieves a rapid inference real-time factor of 0.15 while providing high-quality speech synthesis, capable of zero-shot performance and code-switching, and is trained on a 100K-hour multilingual dataset with resources available for community use.
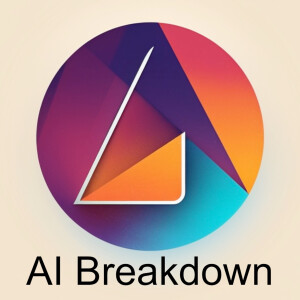
4 days ago
4 days ago
In this episode, we discuss One Initialization to Rule them All: Fine-tuning via Explained Variance Adaptation by Fabian Paischer, Lukas Hauzenberger, Thomas Schmied, Benedikt Alkin, Marc Peter Deisenroth, Sepp Hochreiter. The paper introduces Explained Variance Adaptation (EVA), a method that enhances the fine-tuning of foundation models by using singular value decomposition for a more effective initialization of LoRA matrices. EVA optimizes rank distribution to capture maximum variance before proceeding with task-specific fine-tuning. This improvement leads to faster convergence and better performance across diverse domains such as language, vision, and reinforcement learning.
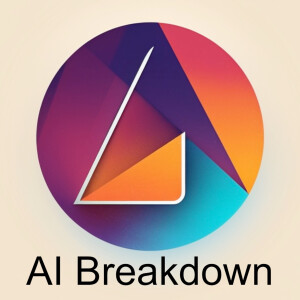
6 days ago
6 days ago
In this episode, we discuss Eliminating Oversaturation and Artifacts of High Guidance Scales in Diffusion Models by Seyedmorteza Sadat, Otmar Hilliges, Romann M. Weber. The paper addresses issues with high guidance scales in classifier-free guidance (CFG) for diffusion models, which can cause oversaturation and artifacts. The authors propose a modified update rule by reducing the influence of the parallel component of the update term, leading to a new method called adaptive projected guidance (APG) that maintains quality without oversaturation at higher guidance scales. APG is effective across various models and improves metrics like FID, recall, and saturation, offering a better alternative to standard CFG.
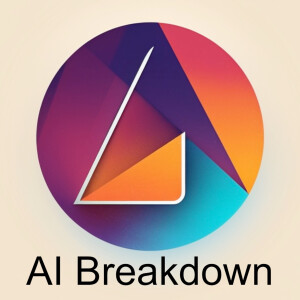
Monday Oct 07, 2024
Monday Oct 07, 2024
In this episode, we discuss NEPTUNE: THE LONG ORBIT TO BENCHMARKING LONG VIDEO UNDERSTANDING by The authors of the paper "NEPTUNE: THE LONG ORBIT TO BENCHMARKING LONG VIDEO UNDERSTANDING" are: - Arsha Nagrani - Mingda Zhang - Ramin Mehran - Rachel Hornung - Nitesh Bharadwaj Gundavarapu - Nilpa Jha - Austin Myers - Xingyi Zhou - Boqing Gong - Cordelia Schmid - Mikhail Sirotenko - Yukun Zhu - Tobias Weyand. The paper introduces "Neptune," a semi-automatic system designed to generate complex question-answer-decoy sets from long video content to enhance comprehension tasks typically limited to short clips. Leveraging large models like Vision-Language Models and Large Language Models, Neptune creates detailed, time-aligned captions and intricate QA sets for videos up to 15 minutes long, aiming to improve annotation efficiency. The dataset emphasizes multimodal reasoning and introduces the GEM metric for evaluating responses, revealing current long video models' weaknesses in understanding temporal and state changes.
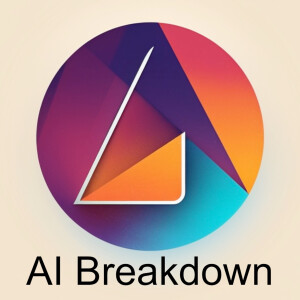
Friday Oct 04, 2024
Friday Oct 04, 2024
In this episode, we discuss SHIC: Shape-Image Correspondences with no Keypoint Supervision by Aleksandar Shtedritski, Christian Rupprecht, Andrea Vedaldi. The paper introduces SHIC, a novel method for learning canonical surface mappings without manual supervision by using foundation models such as DINO and Stable Diffusion. SHIC simplifies the task to image-to-image correspondence prediction, outperforming some supervised techniques. The method uses non-photorealistic template renders to effectively simulate manual annotation, allowing reliable canonical map creation for diverse objects.
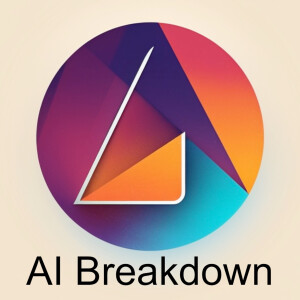
Wednesday Oct 02, 2024
Wednesday Oct 02, 2024
In this episode, we discuss E.T. Bench: Towards Open-Ended Event-Level Video-Language Understanding by Ye Liu, Zongyang Ma, Zhongang Qi, Yang Wu, Ying Shan, Chang Wen Chen. The paper introduces E.T. Bench, a comprehensive benchmark for fine-grained event-level video understanding, evaluating Video-LLMs across 12 tasks and 7K videos. It highlights the challenges these models face in accurately understanding and grounding events within videos. To improve performance, E.T. Chat and an instruction-tuning dataset, E.T. Instruct 164K, are proposed, enhancing models' abilities and underlining the necessity for advanced datasets and models in temporal and multi-event video-language tasks.
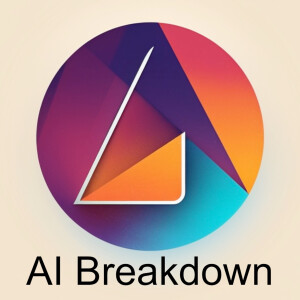
Monday Sep 30, 2024
Monday Sep 30, 2024
In this episode, we discuss LLaVA-3D: A Simple yet Effective Pathway to Empowering LMMs with 3D-awareness by Chenming Zhu, Tai Wang, Wenwei Zhang, Jiangmiao Pang, Xihui Liu. Recent advancements in Large Multimodal Models (LMMs) have significantly improved 2D visual understanding but 3D scene understanding has lagged due to dataset and encoder limitations. The paper introduces LLaVA-3D, a framework that adapts 2D LMMs for 3D understanding by using a 3D Patch representation to link 2D features with 3D positions. This integration allows effective 3D scene understanding without losing 2D capabilities, facilitated by joint 2D and 3D vision-language instruction tuning.
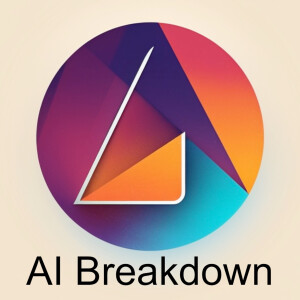
Friday Sep 27, 2024
Friday Sep 27, 2024
In this episode, we discuss DepthCrafter: Generating Consistent Long Depth Sequences for Open-world Videos by Wenbo Hu, Xiangjun Gao, Xiaoyu Li, Sijie Zhao, Xiaodong Cun, Yong Zhang, Long Quan, Ying Shan. DepthCrafter is a novel method for estimating temporally consistent depth in open-world videos without needing additional data like camera poses or optical flow. It generalizes to diverse video content by utilizing a three-stage training strategy rooted in a pre-trained image-to-video diffusion model, enabling it to handle up to 110-frame sequences. Evaluations show DepthCrafter's state-of-the-art performance, bolstering applications like depth-based visual effects and conditional video generation.
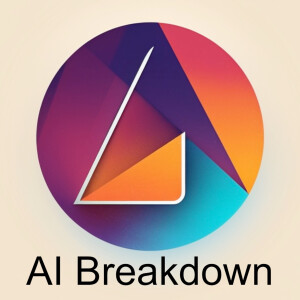
Thursday Sep 26, 2024
Thursday Sep 26, 2024
In this episode, we discuss Programming Every Example: Lifting Pre-training Data Quality like Experts at Scale by Fan Zhou, Zengzhi Wang, Qian Liu, Junlong Li, Pengfei Liu. The paper introduces Programming Every Example (PROX), a framework that enables small language models to refine pre-training corpora by executing fine-grained operations on individual examples, outperforming traditional human-crafted rules. Experimental results show that models trained on PROX-curated data achieve over 2% higher performance across various benchmarks compared to other data selection methods. PROX also significantly enhances domain-specific continual pre-training and reduces training FLOPs, with the authors open-sourcing their data and models for further research.
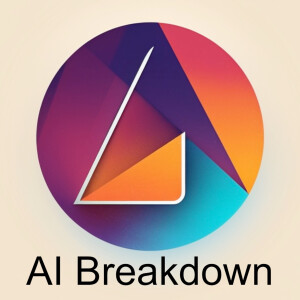
Tuesday Sep 24, 2024
Tuesday Sep 24, 2024
In this episode, we discuss Phantom of Latent for Large Language and Vision Models by Byung-Kwan Lee, Sangyun Chung, Chae Won Kim, Beomchan Park, Yong Man Ro. The paper introduces Phantom, an efficient LLVM family designed to perform comparably to larger models but with significantly smaller sizes, ranging from 0.5B to 7B parameters. By temporarily increasing the latent hidden dimension during multi-head self-attention, Phantom enhances learning capabilities without a substantial increase in model size. Phantom Optimization (PO) combines autoregressive supervised fine-tuning and a direct preference optimization-like concept, resulting in state-of-the-art performance against larger LLVMs.
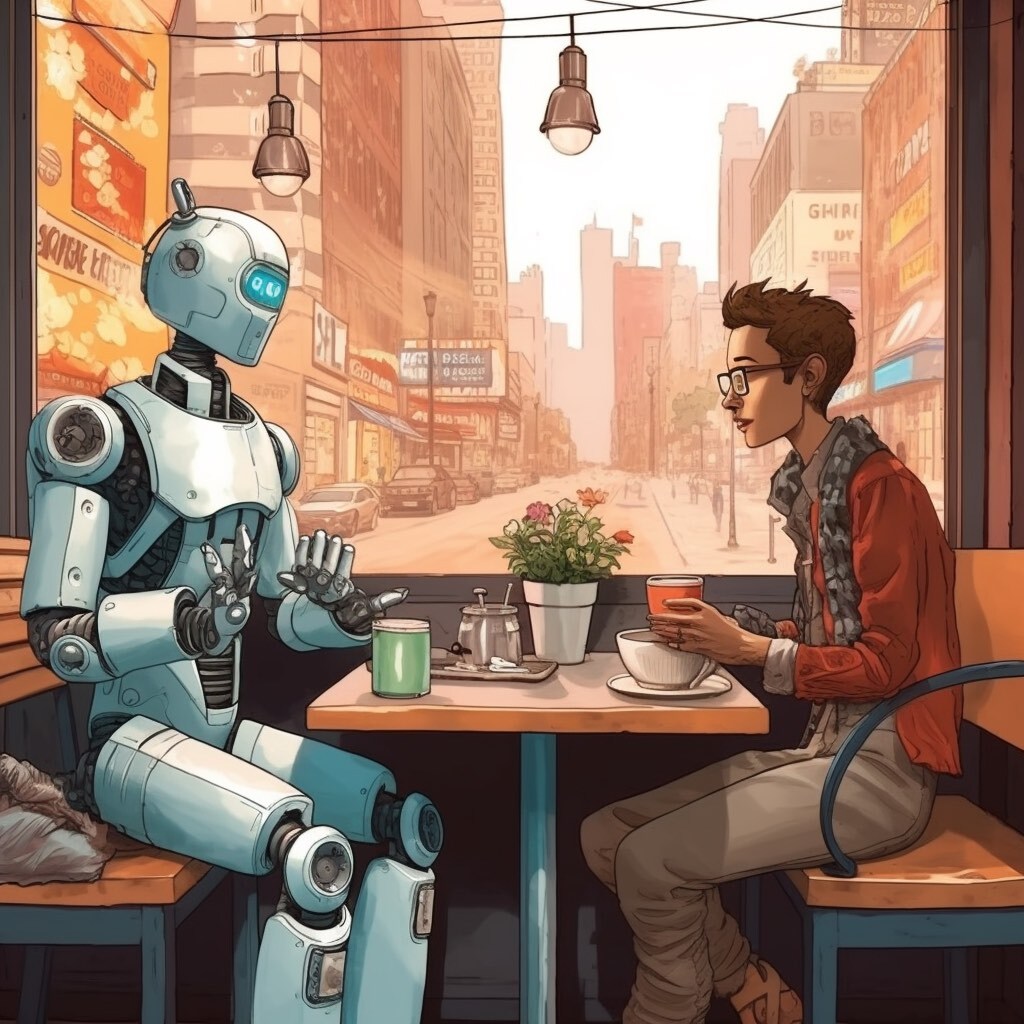
Leverage AI to learn AI
Welcome to the AI Breakdown podcast, where we leverage the power of artificial intelligence to break down recent AI papers and provide simplified explanations of intricate AI topics for educational purposes. We're delighted to have you join us on this exciting journey into the world of artificial intelligence. Our goal is to make complex AI concepts accessible to everyone, and we achieve this by utilizing advanced AI technologies.
Hosts and Ownership: AI Breakdown is under the ownership and management of Megan Maghami and Ramin (Ray) Mehran. Although Megan and Ray lend their voices to the podcast, the content and audio are produced through automated means. Prior to publication, they carefully review the episodes created by AI. They leverage advanced AI technologies, including cutting-edge Large Language Models (LLM) and Text-to-Speech (TTS) systems, to generate captivating episodes. By harnessing these ingenious tools, they deliver enlightening explanations and in-depth analyses on various AI subjects.
Enhancing Your Learning Experience: Your feedback and engagement are crucial to us as we strive to enhance the podcast and provide you with the best possible learning experience. We encourage you to share your thoughts, suggestions, and questions related to our episodes. Together, we can build a vibrant community of AI enthusiasts, learners, and experts, fostering collaboration and knowledge sharing.
Technical Details and Episode Archives: For those interested in the technical aspects behind our AI-generated content, we will provide further insights in upcoming blog posts. Additionally, we will regularly update the blog with published episodes of the AI Breakdown podcast, ensuring convenient access to all our educational resources.